[NIPS 2016 tutorial - Summary] Nuts and bolts of building AI applications using Deep Learning
Deep Learning 2017. 3. 9. 15:27
2017년 3월 9일 목요일
[NIPS 2016 tutorial - Summary] Nuts and bolts of building AI applications using Deep Learning
Today, I am going to review a very educational tutorial by prof. Andrew Ng which was delivered in NIPS 2016. You can download the material by googling though it seems like that there is no official video clip provided from the NIPS 2016 homepage.
Still, you can see the video with almost identical contents (even the title is exactly the same) in the following YouTube link:
* I really recommend you guys to listen to his full lecture. Sometimes, however, watching video takes too much time to get a gist from it. Here, I tried to summarize what he have tried to deliver in his talk. I hope this helps.
** Note that I skipped a few slides or mixed their order to make it easier for me to explain.
The red line which stands for the traditional learning algorithms such as SVM and logistic regression shows a performance plateau after a while with a large amount of data. They did not know what to do with all the data we collected.
For last ten years, due to the rise of internet, mobile and IOT (internet of things), we could march along the X-axis. Andrew Ng commented that this is the number one reason why the DL algorithm works so well.
So... the implication of this :
The second major trend which he is excited about is end-to-end learning.
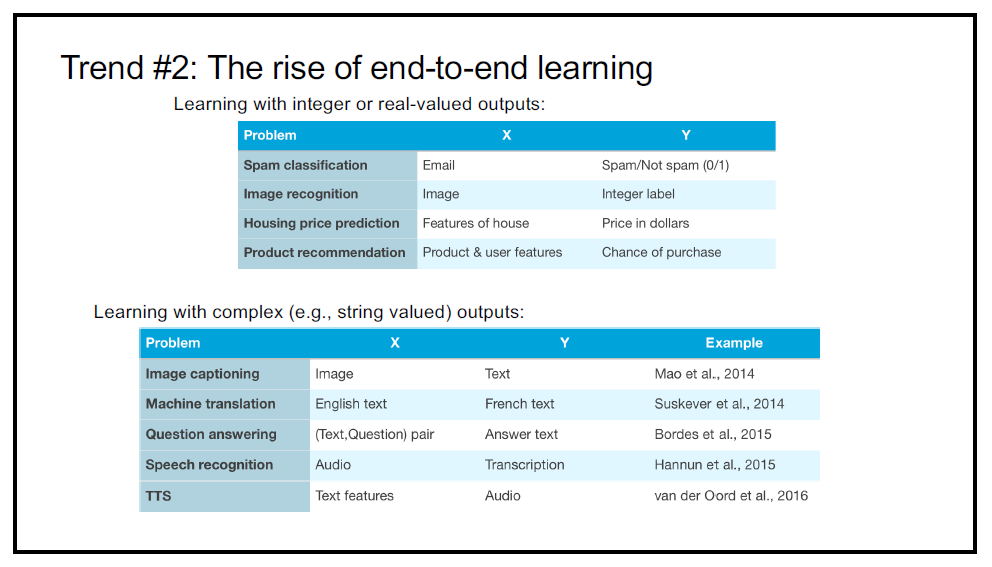
Until recently, a lot of machine learning used real or integer numbers as output. In contrast to those, end-to-end learning can output much more complex things than numbers, e.g. image captioning.
It is called "end-to-end" learning because the input and output of the system are directly linked by a neural network unlike traditional models which have several intermediate steps:
This works well in many cases that are not effective when using traditional models. For example, end-to-end learning shows a better performance in speech recognition tasks.
While presenting this slide, he introduced the following anecdote:
This story seems to say that end-to-end learning is a magic key for any application but rather he warned the audiences that they should be careful when applying the model to their problems.
Despite all the excitements about end-to-end learning, he does not think that this end-to-end learning is the solution for every application. It works well in "some" cases but it does not in many others as well. For example, given the safety-critical requirement of autonomous driving and thus the need for extremely high levels of accuracy, a pure end-to-end approach is still challenging to get to work for autonomous driving.
In addition to this, he also commented that even though DL can almost always train a mapping from X to Y with a reasonable amount of data and you may publish a paper about it, it does not mean that using DL is actually a good idea, e.g. medical diagnosis or imaging.
In the same context, however, I have a slightly different point of view in "phonemes". I think that this can and should be also used as an additional feature in parallel which can reduce the labor of the neural network.
Now let's move on to the next phase of his lecture. Here, he tries to give some sorts of answer or guideline to the following issues:
I think this part is a gist of his lecture. I really really liked his practical tips all of which can be actually applied in my situations right away.
One of those tips he proposed is a kind of "standard workflow" which guides you while training the model:
When the training error is high, this means that the bias between the output of your model and the real data is too big. To mitigate this issue, you need to train longer, use bigger model or adopt a new one. Next, you should check whether your dev error is high or not. If it is, you need more data, try to use some regularization, or use a new model architecture.
* Yes I know, this seems too obvious. Still, I want to mention that everything seems simple once it is organized under an unified system. Constructing an implicit know-how to an explicit framework is not an easy thing.
Here, you should be careful with the implication of the keywords, bias and variance. In his talk, bias and variance have slightly different meanings than textbook definitions (so we do not try to trade off between both entities) although they share a similar concept.
In the era before DL, people used to trade off between the bias and variance by playing with regularization and this coupling was not able to overcome because they were tied too strongly.
Nowadays, however, the coupling between these two seems like to become weaker than before because you can deal with both separately by using simple strategies, i.e. use bigger model (bias) and gather more data (variance).
This also implicitly shows the reason why DL seems to be more applicable to various problems than the traditional learning models. By using DL, there are always at least two ways to solve the problems we are stuck in real life situations as introduced above.
To say whether your error is high or low, you need a reference. Andrew Ng suggests to use a human level error as an optimal error, or Bayes error . He strongly recommended to find the number before going deep in research because this is the very critical component to guide your next step.
Let's say our goal is to build a human level speech system using DL. What we usually do with our data set is to split them with three sets; train, dev(val) and test. Then, the gaps between these errors may occur as below:
You can see the gaps between the errors are named as bias and variance. If you take time and think a while, you will find that it is quite intuitive why he named the gap between human level error and training set error as bias and the other as variance.
If you find that your model has a high bias and a low variance, try to find a new model architecture or simply increase the capacity of the model. On the other hand, if you have a low bias but a high variance, you'd be better to try gathering more data as an easy remedy.
As you can see, because you now use a human level performance as a baseline, you can always have a guideline where to focus on among several options you may have.
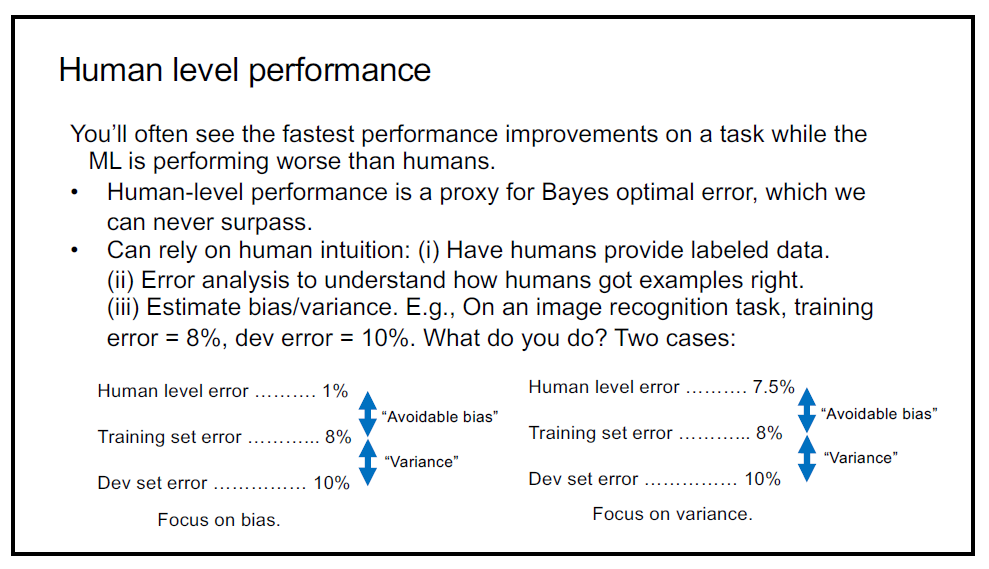
Note that he did not say that it is "easy" to train a big model or to gather a huge amount of data. What he tries to deliver here is that at least you have an "easy option to try" even though you are not an expert in the area. You know... building a new model architecture which actually works is not a trivial task even for the experts.
Still, there remains some unavoidable issues you need to overcome.
To deal with a finite amount of data to efficiently train the model, you need to carefully manipulate the data set or find a way to get more data (data synthesis). Here, I will focus on the former which brings more intuitions for practitioners.
Say you want to build a speech recognition system for a new in-car rearview mirror product. You have 50,000 hours of general speech data and 10 hours of in-car data. How do you split your data?
This is a BAD way to do it:
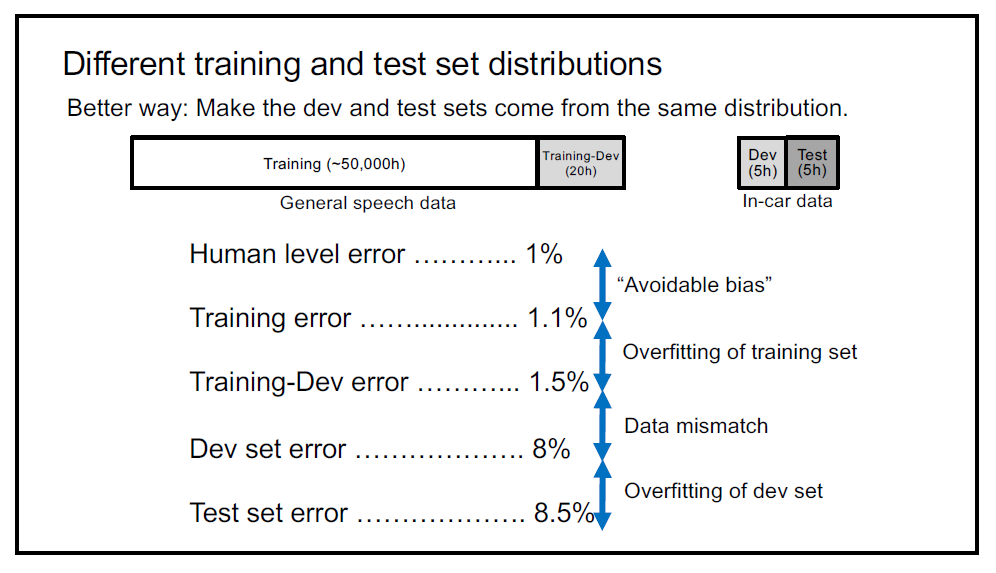
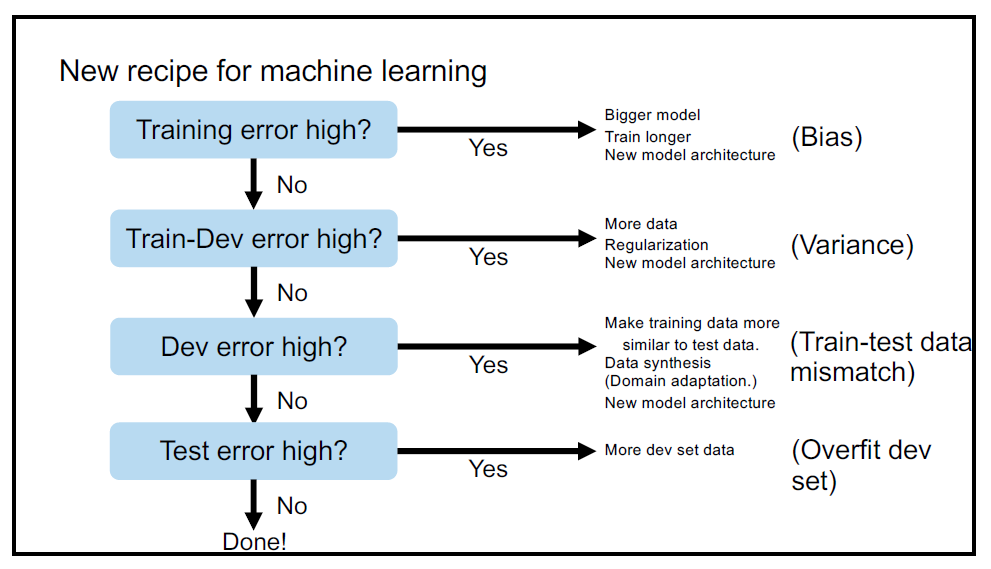
Still, you can see the video with almost identical contents (even the title is exactly the same) in the following YouTube link:
* I really recommend you guys to listen to his full lecture. Sometimes, however, watching video takes too much time to get a gist from it. Here, I tried to summarize what he have tried to deliver in his talk. I hope this helps.
** Note that I skipped a few slides or mixed their order to make it easier for me to explain.
TLDR;
Outline
- Trends of Deep Learning (DL)
- Scale is driving DL progress
- Rise of end-to-end learning
- When to and when not to use "End-to-End" learning
- Machine Learning (ML) Strategy (very practical advice)
- How to manage train/dev/test data set and bias/variance
- Basic recipe for ML
- Defining a human level performance of each application is very useful
- Scale is driving DL progress
- Rise of end-to-end learning
- When to and when not to use "End-to-End" learning
- How to manage train/dev/test data set and bias/variance
- Basic recipe for ML
- Defining a human level performance of each application is very useful
Trend #1
Q) Why is Deep Learning working so well NOW?
A) Scale drives DL progress
The red line which stands for the traditional learning algorithms such as SVM and logistic regression shows a performance plateau after a while with a large amount of data. They did not know what to do with all the data we collected.
For last ten years, due to the rise of internet, mobile and IOT (internet of things), we could march along the X-axis. Andrew Ng commented that this is the number one reason why the DL algorithm works so well.
So... the implication of this :
To hit the top margin, you need a huge amount of data and a large NN model.
Trend #2
The second major trend which he is excited about is end-to-end learning.
Until recently, a lot of machine learning used real or integer numbers as output. In contrast to those, end-to-end learning can output much more complex things than numbers, e.g. image captioning.
It is called "end-to-end" learning because the input and output of the system are directly linked by a neural network unlike traditional models which have several intermediate steps:
This works well in many cases that are not effective when using traditional models. For example, end-to-end learning shows a better performance in speech recognition tasks.
While presenting this slide, he introduced the following anecdote:
"This end-to-end story really upset many people. I used to get around and say that I believe "phonemes" are the fantasy of the linguists and machines can do well without them. One day at the meeting in Stanford a linguist yelled at me in public for saying that. Well...we turned out to be right."
This story seems to say that end-to-end learning is a magic key for any application but rather he warned the audiences that they should be careful when applying the model to their problems.
Despite all the excitements about end-to-end learning, he does not think that this end-to-end learning is the solution for every application. It works well in "some" cases but it does not in many others as well. For example, given the safety-critical requirement of autonomous driving and thus the need for extremely high levels of accuracy, a pure end-to-end approach is still challenging to get to work for autonomous driving.
In addition to this, he also commented that even though DL can almost always train a mapping from X to Y with a reasonable amount of data and you may publish a paper about it, it does not mean that using DL is actually a good idea, e.g. medical diagnosis or imaging.
End-to-End works only when you have enough (x,y) data to learn function of needed level of complexity.I totally agree with his point that we should not naively rely on the learning capability of the neural network. We should exploit all the power and knowledge of hand-designed or carefully chosen features which we already have.
In the same context, however, I have a slightly different point of view in "phonemes". I think that this can and should be also used as an additional feature in parallel which can reduce the labor of the neural network.
Machine Learning Strategy
Now let's move on to the next phase of his lecture. Here, he tries to give some sorts of answer or guideline to the following issues:
- Often you will have a lot of ideas for how to improve an AI system, what do you do?
- Good strategy will help avoid months of wasted effort. Then, what is it?
I think this part is a gist of his lecture. I really really liked his practical tips all of which can be actually applied in my situations right away.
One of those tips he proposed is a kind of "standard workflow" which guides you while training the model:
When the training error is high, this means that the bias between the output of your model and the real data is too big. To mitigate this issue, you need to train longer, use bigger model or adopt a new one. Next, you should check whether your dev error is high or not. If it is, you need more data, try to use some regularization, or use a new model architecture.
* Yes I know, this seems too obvious. Still, I want to mention that everything seems simple once it is organized under an unified system. Constructing an implicit know-how to an explicit framework is not an easy thing.
Here, you should be careful with the implication of the keywords, bias and variance. In his talk, bias and variance have slightly different meanings than textbook definitions (so we do not try to trade off between both entities) although they share a similar concept.
In the era before DL, people used to trade off between the bias and variance by playing with regularization and this coupling was not able to overcome because they were tied too strongly.
Nowadays, however, the coupling between these two seems like to become weaker than before because you can deal with both separately by using simple strategies, i.e. use bigger model (bias) and gather more data (variance).
This also implicitly shows the reason why DL seems to be more applicable to various problems than the traditional learning models. By using DL, there are always at least two ways to solve the problems we are stuck in real life situations as introduced above.
Human Level Error as a Reference
To say whether your error is high or low, you need a reference. Andrew Ng suggests to use a human level error as an optimal error, or Bayes error . He strongly recommended to find the number before going deep in research because this is the very critical component to guide your next step.
Let's say our goal is to build a human level speech system using DL. What we usually do with our data set is to split them with three sets; train, dev(val) and test. Then, the gaps between these errors may occur as below:
You can see the gaps between the errors are named as bias and variance. If you take time and think a while, you will find that it is quite intuitive why he named the gap between human level error and training set error as bias and the other as variance.
If you find that your model has a high bias and a low variance, try to find a new model architecture or simply increase the capacity of the model. On the other hand, if you have a low bias but a high variance, you'd be better to try gathering more data as an easy remedy.
As you can see, because you now use a human level performance as a baseline, you can always have a guideline where to focus on among several options you may have.
Note that he did not say that it is "easy" to train a big model or to gather a huge amount of data. What he tries to deliver here is that at least you have an "easy option to try" even though you are not an expert in the area. You know... building a new model architecture which actually works is not a trivial task even for the experts.
Still, there remains some unavoidable issues you need to overcome.
Data Crisis
To deal with a finite amount of data to efficiently train the model, you need to carefully manipulate the data set or find a way to get more data (data synthesis). Here, I will focus on the former which brings more intuitions for practitioners.
Say you want to build a speech recognition system for a new in-car rearview mirror product. You have 50,000 hours of general speech data and 10 hours of in-car data. How do you split your data?
This is a BAD way to do it:
Having a mismatched dev and test distributions is not a good idea. You may spend months optimizing for dev set performance only to find it does not work well on the test set.
So the following is his suggestion to do better:
A few remaining remarks
While the performance is worse than humans, there are many good ways to progress;
- error analysis
- estimate bias/variance
- etc.
After surpassing the human performance or at least near the point, however, what you usually observe is that the progress becomes slow and almost stuck. There can be several reasons such as:
- Label is made by human (so the limit lies here)
- Maybe because human level error is close to the optimal error (Bayes error)
What you can do here is to find a subset of data that still works worse than human and make the model do better.
I hope you enjoyed my summary. Thank you for reading :)
Interesting references
'Deep Learning' 카테고리의 다른 글
스마트폰에서 딥러닝 실행방법-Squeezing Deep Learning into Mobile Phones - A Practitioner's guide (0) | 2017.03.18 |
---|---|
Winning Tips on Machine Learning Competitions by Kazanova, Current Kaggle #3 | HackerEarth Blog (0) | 2017.03.13 |
Android에서 TensorFlow 실행하기 (0) | 2017.03.09 |
Fully Convolutional Networks (FCNs) for Image Segmentation (0) | 2017.02.02 |
무료 e-러닝 강좌 머신러닝을 이용한 주식 트레이딩 (0) | 2017.01.31 |