Free and Easy to use online image effects. Try the Perspective effect.
www4.lunapic.com
빨간색 점 4개를 마우스로 드래그하면 원하는 사각형을 만들 수 있다.
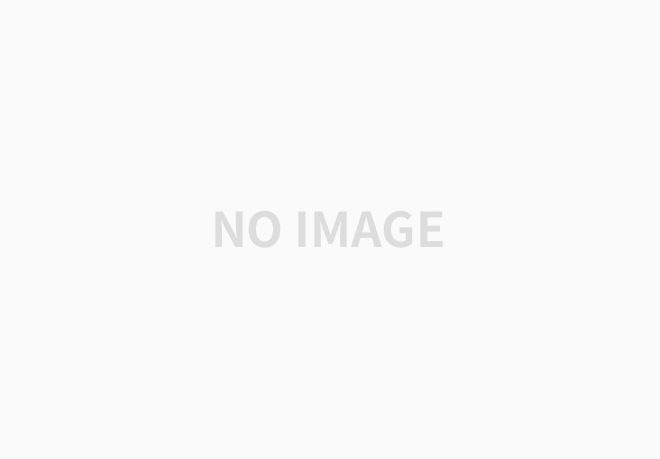
사진 설명을 입력하세요.
Free and Easy to use online image effects. Try the Perspective effect.
www4.lunapic.com
빨간색 점 4개를 마우스로 드래그하면 원하는 사각형을 만들 수 있다.
사진 설명을 입력하세요.
vgg = vgg16.VGG16(include_top=False, weights='imagenet', input_shape=(224, 224, 3))
단일 컬러영상(3채널)을 입력시킬 때는 보통 위와 같이 vgg16아키텍처를 불러오고 이미지넷 웨이트도 복사해온다.
아래 코드는 3채널이 아닌 영상의 아키텍처를 불러올 때 사용한다.
vgg = vgg16.VGG16(include_top=False, weights=None, input_shape=(224, 224, 6))
위 코드는 vgg16의 아키텍처의 입력영상의 채널수가 6일 경우이다. 이렇게 하면 에러가 나지 않고 아키텍처가 생성된다. weights=None이라고 입력해주는 게 중요하다. 이 옵션을 넣지 않으면 에러가 발생한다. 대신 weights=None을 설정하면 imagenet에서 학습된 웨이트는 복사되지 않는다. 아래의 레이어 정보를 보면 입력영상의 채널이 6개이다. 0번 레이어만 shape이 (채널수가) 다르고 나머지 레이어는 원래 vgg16과 같은 shape의 레이어들이다.
넘파이의 3차원에서 axis는 아래 그림과 같이 axis=0은 깊이, axis=1은 세로축, axis=2는 가로축이다.
a = np.array([[1, 2], [3, 4]])
b = np.array([[5, 6], [7, 8]])
일 때
1. np.stack((a, b), axis=0)
np.stack((a, b), axis=0)
# array([[[1, 2],
# [3, 4]],
# [[5, 6],
# [7, 8]]])
그림은 아래와 같다. axis=0이므로 reshape에서 1번째에 1이 추가된다.
2. np.stack((a, b), axis=1)
np.stack((a, b), axis=1)
# array([[[1, 2],
# [5, 6]],
# [[3, 4],
# [7, 8]]])
그림은 아래와 같다. axis=1이므로 reshape에서 2번째에 1이 추가된다.
3. np.stack((a, b), axis=2)
np.stack((a, b), axis=2)
# array([[[1, 5],
# [2, 6]],
# [[3, 7],
# [4, 8]]])
그림은 아래와 같다. axis=2이므로 reshape에서 3번째에 1이 추가된다.
참고로, 아래 그림은 np.reshape을 이용해 shape=(2,3,2) --> shape=(2,3,2)으로 바뀌는 과정을 나타낸다. 가장 바깥쪽(3번째 axis)이 먼저 unrolling되고, rolling될 때도 가장 바깥쪽(3번째 axis)이 먼저 rolling된다. (https://towardsdatascience.com/np-reshape-in-python-39b4636d7d91 참조)
[self][Python NumPy] 배열을 수평(옆)으로, 수직(위아래)으로 붙이기 : np.r_, np.c_ (0) | 2020.09.18 |
---|
https://stackoverflow.com/questions/63746066/installing-cusignal-on-windows-10 조언대로
git clone https://github.com/rapidsai/cusignal.git
cd cusignal
build.sh
실행하니 해결됨.
'pip install cupy'로 설치되지 않을 때 (0) | 2023.03.02 |
---|---|
cusolver64_10.dll not found만 찾을 수 없다는 에러 (0) | 2021.03.16 |
tensorflow-gpu설치하다 다음의 오류났다 (0) | 2021.03.16 |
안녕하세요, 왕초보입니다. MacOS, Windows 또는 LInux ? 인공지능을 제대로 공부하려는데요, 어떤 운영체제에서 시작하는 것이 성 (0) | 2020.08.26 |
https://lambdalabs.com (한국에 판매를 하는지는 모르겠지만..) 이 사이트에서 여러가지 구성 만들어보고 대략적인 가격도 확인 (0) | 2020.06.05 |
'pip install cupy'로 설치하려니 설치되지 않고 계속 진행중이라고만 나온다.
검색해보니 https://twitter.com/mitmul/status/986171511873523712?lang=en 및 https://github.com/cupy/cupy/issues/1643#issuecomment-420896839, https://docs.cupy.dev/en/latest/install.html#install-cupy
에서 'pip install cupy'로 설치가 안되면 'pip install cupy-cuda112' (cuda버전이 11.2일때) 이런식으로 설치해보라해서 하니 설치됨.
cuda버전 확인방법은 윈도우에서는 명령프롬프트 창에 'nvcc --version'
pip install cusignal 에러 시 설치방법 (0) | 2023.03.02 |
---|---|
cusolver64_10.dll not found만 찾을 수 없다는 에러 (0) | 2021.03.16 |
tensorflow-gpu설치하다 다음의 오류났다 (0) | 2021.03.16 |
안녕하세요, 왕초보입니다. MacOS, Windows 또는 LInux ? 인공지능을 제대로 공부하려는데요, 어떤 운영체제에서 시작하는 것이 성 (0) | 2020.08.26 |
https://lambdalabs.com (한국에 판매를 하는지는 모르겠지만..) 이 사이트에서 여러가지 구성 만들어보고 대략적인 가격도 확인 (0) | 2020.06.05 |
대용량 파일 다운 받는 중 끊기면 처음부터 다시 받아야하는 번거로움이 있다. 이때 쓰면 좋은 코드.
여러 세그멘트 단위로 분할하여 다운받는 방식인듯.
https://github.com/spaceromany/resume_download_for_scamps
resume_download_for_scamps
SCAMPS (https://github.com/danmcduff/scampsdataset) consists of many video files. But download URL link does not provide resume download support. Our code is for downloading SCAMPS dataset using python and provides resume function.
https://www.facebook.com/groups/TensorFlowKR/posts/1868875980120118/
[rgb이미지의 2d 콘볼루션은 3d콘볼루션으로 작용한다][convolution kernel의 깊이(depth)는 생략되어 있다]convolution 연산에서 입력영상의 컬러채널 또는 다수의 깊이(depth channel)채널과 필터의 콘볼루.. (0) | 2021.04.15 |
---|---|
NVIDIA Research Unveils Flowtron, an Expressive and Natural Speech Synthesis Mod (0) | 2020.05.15 |
수학을 포기한 직업 프로그래머가 머신러닝 학습을 시작하기위한 학습법 소개 (0) | 2017.07.27 |
파이썬 쥬피터를 이용한 텐서플로우 개발환경 구성하기 (0) | 2017.04.13 |
Why Momentum Really Works (0) | 2017.04.05 |
box plot은 사분위수(상위 25%, 50%, 75%를 각각 Q1, Q2, Q3 표현)를 그림으로 나타낸 것이다. Q1 ~ Q3 사이의 상위 25~75% 구간이 가운데 box로 나타내지고, Q2(상위 50%)는 박스 중간에 직선으로 표시된다. Q1 - (Q3 - Q1) * 1.5 이하와 Q3 + (Q3 - Q1) * 1.5 이상의 구간에 존재하는 샘플들은 이상치(outlier)로 간주되며 점으로 표시된다. 이 범위 내의 최소값(minimum)과 최대값(maximum)을 양 끝의 수직선으로 나타낸다.
아래 코드는 https://stackoverflow.com/questions/50554235/how-to-base-seaborn-boxplot-whiskers-on-percentiles 의 코드를 수정한 것인데, seaborn box plot을 이해하기에 좋은 예제인 것 같다.
import seaborn as sns
import matplotlib.pyplot as plt
tips = sns.load_dataset("tips")
a1 = tips["total_bill"]
ax = sns.boxplot(x=tips["total_bill"], whis=[5, 95])
plt.grid(True)
plt.figure()
ax = sns.boxplot(x=tips["total_bill"])
plt.grid(True)
print(tips["total_bill"].describe())
실행결과
[229 rows x 16 columns]
count 244.000000
mean 19.785943
std 8.902412
min 3.070000
25% 13.347500
50% 17.795000
75% 24.127500
max 50.810000
Name: total_bill, dtype: float64
아래 그림은 5%와 95%를 minimum, maximum으로 구분한 플롯.
아래 그림은 원래 함수의 디폴트에 따라 minimum, maximum으로 구분한 플롯.
https://jimmy-ai.tistory.com/51에서는
"참고로, box plot은 사분위수(상위 25, 50, 75% 숫자 : Q1, Q2, Q3)를 기준으로
Q1 ~ Q3 사이의 상위 25~75% 구간이 가운데 box로 색칠되고,
Q1 - (Q3 - Q1) * 1.5 이하와 Q3 + (Q3 - Q1) * 1.5 이상의 구간을 끝 선으로 나타내고
이 밖의 범위에 해당하는 숫자는 이상치(점으로 표시)로 취급하는 그림입니다.
박스의 끝점이 Q1, Q3에 해당하고, 박스 가운데 선이 Q2에 해당하는 것을 참고하세요."
라고 설명하고 있다. 그런데, Q1 - (Q3 - Q1) * 1.5 이하와 Q3 + (Q3 - Q1) * 1.5 이상의 구간을 끝 선으로 나타낸다면, 박스로부터 양 끝으로부터 양 끝 선(수직선)까지 길이가 같아야하는데 다르다. 그래서 검색해보니,
https://datagy.io/seaborn-boxplot/ 에서 제대로 설명하고 있다.
위 그림은 https://flowingdata.com/2008/02/15/how-to-read-and-use-a-box-and-whisker-plot/ 에서 설명하고 있는 그림인데, minumum을 25%의 1.5배보다 작은 것이라고 설명하고 있는데 잘못 설명하여 헷갈리게 만든다.
https://stackoverflow.com/questions/51748514/does-imagedatagenerator-add-more-images-to-my-dataset
Does ImageDataGenerator add more images to my dataset?
I'm trying to do image classification with the Inception V3 model. Does ImageDataGenerator from Keras create new images which are added onto my dataset? If I have 1000 images, will using this funct...
stackoverflow.com
I'm trying to do image classification with the Inception V3 model. Does ImageDataGenerator from Keras create new images which are added onto my dataset? If I have 1000 images, will using this function double it to 2000 images which are used for training? Is there a way to know how many images were created and now fed into the model?
Short answer: 1) All the original images are just transformed (i.e. rotation, zooming, etc.) every epoch and then used for training, and 2) [Therefore] the number of images in each epoch is equal to the number of original images you have.
| 컴퓨터공학 | 컴퓨터/IT | 국내도서 | 베스트셀러 - 교보문고
의 다양한 도서를 확인해 보세요. 판매인기순 및 새로나온 순으로 도서들을 확인할 수 있습니다.
kyobobook.co.kr
순위
|
바코드
|
도서명
|
저자명
|
출판사명
|
출간일
|
정가
|
|
||||||
1
|
9791160507966
|
머신 러닝 교과서 with 파이썬, 사이킷런, 텐서플로
|
세바스찬 라시카
|
길벗
|
20190524
|
33,000
|
2
|
9788968484636
|
밑바닥부터 시작하는 딥러닝
|
사이토 고키
|
한빛미디어
|
20170103
|
24,000
|
3
|
9791162241745
|
밑바닥부터 시작하는 딥러닝 2
|
사이토 고키
|
한빛미디어
|
20190501
|
29,000
|
4
|
9791162241974
|
김기현의 자연어 처리 딥러닝 캠프: 파이토치 편(소문난 명강의)
|
김기현
|
한빛미디어
|
20190701
|
38,000
|
5
|
9791160505979
|
케라스 창시자에게 배우는 딥러닝(Deep Learning with Python)
|
프랑소와 숄레
|
길벗
|
20181022
|
33,000
|
6
|
9791189909024
|
실체가 손에 잡히는 딥러닝, 기초부터 실전 프로그래밍
|
아즈마 유키나가
|
책만
|
20190618
|
26,000
|
7
|
9791162241646
|
파이썬 라이브러리를 활용한 머신러닝
|
안드레아스 뮐러
|
한빛미디어
|
20190329
|
32,000
|
8
|
9791162240731
|
핸즈온 머신러닝
|
오렐리앙 제롱
|
한빛미디어
|
20180427
|
33,000
|
9
|
9791160503715
|
모두의 딥러닝
|
조태호
|
길벗
|
20171227
|
24,000
|
10
|
9788965402282
|
인공지능을 위한 수학
|
이시카와 아키히코
|
프리렉
|
20181122
|
25,000
|
11
|
9791162241899
|
파이토치 첫걸음
|
최건호
|
한빛미디어
|
20190607
|
23,000
|
12
|
9788931458398
|
텐서플로로 배우는 딥러닝
|
솔라리스
|
영진닷컴
|
20181116
|
26,000
|
13
|
9791162240441
|
처음 배우는 딥러닝 수학
|
와쿠이 요시유키
|
한빛미디어
|
20180201
|
25,000
|
14
|
9791156641582
|
기계 학습
|
오일석
|
한빛아카데미
|
20171205
|
32,000
|
15
|
9791161752594
|
PyTorch로 시작하는 딥러닝
|
비슈누 수브라마니안
|
에이콘출판
|
20190218
|
25,000
|
16
|
9791188621255
|
패턴 인식과 머신 러닝(제이펍의 인공지능 시리즈 11)
|
크리스토퍼 비숍
|
제이펍
|
20180910
|
46,000
|
17
|
9791158391379
|
텐서플로와 머신러닝으로 시작하는 자연어 처리(데이터 사이언스 시리즈 30)
|
전창욱
|
위키북스
|
20190215
|
30,000
|
18
|
9791158390723
|
파이썬과 케라스로 배우는 강화학습(위키북스 데이터 사이언스 시리즈 4)
|
이웅원
|
위키북스
|
20170731
|
27,000
|
19
|
9791158391287
|
강화학습 /심층강화학습 실전 입문(PyTorch를 활용한)(위키북스 데이터 사이언스 시리즈 25)
|
오가와 유타로
|
위키북스
|
20181218
|
25,000
|
20
|
9791188621422
|
심층 학습(제이펍의 인공지능 시리즈 13)
|
이안 굿펠로
|
제이펍
|
20181031
|
42,000
|
21
|
9791158390679
|
파이썬을 이용한 머신러닝, 딥러닝 실전 개발 입문(데이터 사이언스 시리즈 3)
|
쿠지라 히코우즈쿠에
|
위키북스
|
20170615
|
30,000
|
22
|
9791190014366
|
딥러닝에 목마른 사람들을 위한 PyTorch
|
장지수
|
비제이퍼블릭
|
20190628
|
24,000
|
23
|
9788931588057
|
머신러닝(만화로 쉽게 배우는)
|
아라키 마사히로
|
성안당
|
20190522
|
15,000
|
24
|
9788960882133
|
파이썬 딥러닝 케라스(블록과 함께 하는)
|
김태영
|
디지털북스
|
20170930
|
25,000
|
25
|
9791158390914
|
기초 수학으로 이해하는 머신러닝 알고리즘(데이터 사이언스 시리즈 10)
|
타테이시 켄고
|
위키북스
|
20180213
|
22,000
|
26
|
9791161752006
|
컴퓨터 비전과 딥러닝
|
라쟈링가파 샨무갸마니
|
에이콘출판
|
20180831
|
30,000
|
27
|
9791185890418
|
인공지능 1: 현대적 접근방식(3판)
|
스튜어트 러셀
|
제이펍
|
20160129
|
36,000
|
28
|
9791162240137
|
골빈해커의 3분 딥러닝 텐서플로맛
|
김진중
|
한빛미디어
|
20170925
|
22,000
|
29
|
9791158391065
|
파이썬과 케라스를 이용한 딥러닝/강화학습 주식투자(위키북스 데이터 사이언스 시리즈 15)
|
김문권
|
위키북스
|
20180529
|
25,000
|
30
|
9791158391225
|
PyTorch를 활용한 머신러닝, 딥러닝 철저 입문(위키북스 데이터 사이언스 시리즈 22)
|
코이즈미 사토시
|
위키북스
|
20181107
|
25,000
|
31
|
9791158391478
|
파이썬을 이용한 머신러닝, 딥러닝 실전 앱 개발(위키북스 데이터 사이언스 시리즈 32)
|
쿠지라 히코우즈쿠에
|
위키북스
|
20190328
|
27,000
|
32
|
9791161751283
|
R로 마스터하는 머신 러닝 2/e(2판)(acorn+Packt 시리즈)
|
코리 레즈마이스터
|
에이콘출판
|
20180309
|
36,000
|
33
|
9791162241387
|
기계는 어떻게 생각하고 학습하는가
|
뉴 사이언티스트
|
한빛미디어
|
20181205
|
17,000
|
34
|
9791164260560
|
빅데이터 & 인공지능 with 생물정보학(애프터스킬)
|
양우진
|
아이콕스
|
20190630
|
22,000
|
35
|
9791185459547
|
마스터 알고리즘
|
페드로 도밍고스
|
비즈니스북스
|
20160730
|
22,000
|
36
|
9791185890425
|
인공지능 2: 현대적 접근방식(3판)
|
스튜어트 러셀
|
제이펍
|
20160129
|
36,000
|
37
|
9791188621460
|
케라스 창시자의 딥러닝 with R(제이팝의 인공지능 시리즈 15)
|
프랑소와 숄레
|
제이펍
|
20190221
|
29,000
|
38
|
9791160506013
|
데이터 분석을 위한 머신 러닝 입문
|
하시모토 타이이치
|
길벗
|
20181105
|
22,000
|
39
|
9788970859040
|
패턴 인식
|
오일석
|
교보문고
|
20080820
|
25,000
|
40
|
9788970939261
|
텐서플로우 입문(인공지능을 위한)
|
김유두
|
광문각
|
20190125
|
16,000
|
41
|
9788970939445
|
구글 딥러닝 프레임워크: 텐서플로우 실전(예제로 플어보는)
|
정저위
|
광문각
|
20190525
|
28,000
|
42
|
9791158391300
|
나의 첫 머신러닝/딥러닝(데이터 사이언스 시리즈 27)
|
허민석
|
위키북스
|
20190110
|
26,000
|
43
|
9791162240809
|
인공지능 첫걸음(아무것도 모르고 시작하는)
|
히가시나카 류이치로
|
한빛미디어
|
20180601
|
22,000
|
44
|
9788960778665
|
파이썬으로 배우는 인공지능(acorn+PACKT 시리즈)
|
프라틱 조쉬
|
에이콘출판
|
20170524
|
40,000
|
45
|
9788968484902
|
텐서플로 첫걸음
|
조르디 토레스
|
한빛미디어
|
20160829
|
16,000
|
46
|
9788970509594
|
인공지능
|
이건명
|
생능출판
|
20180903
|
35,000
|
47
|
9791158391232
|
텐서플로 딥러닝 프로젝트(실전활용!)(위키북스 데이터 사이언스 시리즈 24)
|
루카 마사론
|
위키북스
|
20181121
|
27,000
|
48
|
9791161752426
|
피처 엔지니어링, 제대로 시작하기
|
앨리스 젱
|
에이콘출판
|
20190102
|
25,000
|
49
|
9791162241240
|
파이썬으로 배우는 머신러닝의 교과서
|
이토 마코토
|
한빛미디어
|
20181101
|
29,000
|
50
|
9791185890982
|
인공지능 70(제이펍의 인공지능 시리즈 6)
|
미야케 요이치로
|
제이펍
|
20170724
|
13,000
|
51
|
9791188621590
|
파이토치 첫걸음(아이러브A.I. 16)
|
두세교
|
제이펍
|
20190509
|
24,000
|
52
|
9791195581191
|
머신러닝 쉽게 이해하기
|
에템 알페이딘
|
유엑스리뷰(UX REVIEW)
|
20180323
|
32,000
|
53
|
9788931459531
|
생활을 변화시키는 인공지능
|
다쿠치 카즈히로
|
영진닷컴
|
20181103
|
15,000
|
54
|
9788955027648
|
인공지능(Python과 TensorFlow로 구현한)(개정판)
|
이권윤
|
글로벌
|
20180903
|
17,000
|
55
|
9788957272664
|
영상처리 기반의 인공지능 입문
|
고병철
|
그린
|
20180521
|
18,000
|
56
|
9788965402237
|
머신러닝 딥러닝 바로가기
|
요시카와 하야토
|
프리렉
|
20180724
|
25,000
|
57
|
9788968487323
|
딥러닝 첫걸음
|
김성필
|
한빛미디어
|
20161230
|
18,000
|
58
|
9788997924387
|
1억배 빠른 양자 컴퓨터가 온다(누구나 읽을 수 있는 IT 과학이야기 3)
|
니시모리 히데토시
|
로드북
|
20180416
|
15,000
|
59
|
9791156644538
|
텐서플로로 배우는 딥러닝(IT CookBook 256)
|
박혜정
|
한빛아카데미
|
20190630
|
27,000
|
60
|
9791158390624
|
인공지능, 쉽게 이해하고 넓게 활용하기(최신)
|
칸자키 요지
|
위키북스
|
20170407
|
15,000
|
61
|
9791158391256
|
유니티 ML-Agents(따라 하면서 배우는)(위키북스 데이터 사이언스 시리즈 23)
|
마이클 랜햄
|
위키북스
|
20181121
|
22,000
|
62
|
9791158391393
|
실전! 딥러닝(데이터 사이언스 시리즈 29)
|
오타 미쯔히사
|
위키북스
|
20190215
|
25,000
|
63
|
9791159712111
|
인공지능의 세계(포스트 사이언스(Post Science) 2)
|
고자키 요지
|
북스힐
|
20190605
|
13,000
|
64
|
9791161750347
|
C++와 CUDA C로 구현하는 딥러닝 알고리즘 세트(에이콘 데이터 과학 시리즈)(전3권)
|
티모시 마스터즈
|
에이콘출판
|
20170818
|
78,000
|
65
|
9791161752334
|
실전 금융 머신러닝 완벽 분석(에이콘 금융 퀀트 머신러닝 융합 시리즈)
|
마르코스 로페즈 데 프라도
|
에이콘출판
|
20190102
|
40,000
|
66
|
9791162240816
|
머신러닝 실무 프로젝트
|
아리가 미치아키
|
한빛미디어
|
20180605
|
18,000
|
67
|
9791187431152
|
딥다 딥러닝
|
양지헌
|
스포트라잇북
|
20180830
|
30,000
|
교보문고 인공지능분야 국내도서 베스트셀러 최근1주일기준 (2022년 7월 13일) (0) | 2022.07.13 |
---|---|
Amazon에서 MXNet 기반으로 작성한 유명한 텍스트북 Dive Into Deep Learning 에 PyTorch 버전의 코드들이 수록된 (0) | 2020.07.08 |
9 Best AI & Machine Learning Books To Read In 2020 (0) | 2020.04.24 |
한빛미디어 AI 서적들 (0) | 2020.01.08 |
교보문고 2019 IT 전문서 올해의 책 (0) | 2020.01.08 |
순위
|
바코드
|
도서명
|
저자명
|
출판사명
|
출간일
|
정가
|
인공지능
|
||||||
1
|
9791162243664
|
혼자 공부하는 머신러닝+딥러닝
|
박해선
|
한빛미디어
|
20201221
|
26,000
|
2
|
9788968484636
|
밑바닥부터 시작하는 딥러닝
|
사이토 고키
|
한빛미디어
|
20170103
|
24,000
|
3
|
9791165218942
|
딥러닝 파이토치 교과서
|
서지영
|
길벗
|
20220304
|
36,000
|
4
|
9791162241745
|
밑바닥부터 시작하는 딥러닝 2
|
사이토 고키
|
한빛미디어
|
20190501
|
29,000
|
5
|
9791191905137
|
데싸노트의 실전에서 통하는 머신러닝(Must Have)
|
권시현
|
골든래빗(주)
|
20220708
|
34,000
|
6
|
9791162242964
|
핸즈온 머신러닝(2판)
|
오렐리앙 제롱
|
한빛미디어
|
20200504
|
55,000
|
7
|
9791162243596
|
밑바닥부터 시작하는 딥러닝 3
|
사이토 고키
|
한빛미디어
|
20201110
|
38,000
|
8
|
9791163033165
|
Do it! BERT와 GPT로 배우는 자연어 처리
|
이기창
|
이지스퍼블리싱
|
20211201
|
20,000
|
9
|
9791163033790
|
인공지능은 게임을 어떻게 움직이는가?
|
미야케 요이치로
|
이지스퍼블리싱
|
20220630
|
18,000
|
10
|
9791165219246
|
모두의 딥러닝(3판)
|
조태호
|
길벗
|
20220331
|
24,000
|
11
|
9791160505979
|
케라스 창시자에게 배우는 딥러닝(Deep Learning with Python)
|
프랑소와 숄레
|
길벗
|
20181022
|
33,000
|
12
|
9791189909413
|
파이토치 딥러닝 마스터
|
엘리 스티븐스
|
책만
|
20220622
|
35,000
|
13
|
9791162244852
|
구글 BERT의 정석
|
수다르산 라비찬디란
|
한빛미디어
|
20211103
|
34,000
|
14
|
9791158393168
|
텐서플로 2와 머신러닝으로 시작하는 자연어 처리(2판)(위키북스 데이터 사이언스 시리즈 79)
|
전창욱
|
위키북스
|
20220324
|
36,000
|
15
|
9791161756578
|
고객 리텐션의 전략(데이터 과학)
|
칼 골드
|
에이콘출판
|
20220630
|
40,000
|
16
|
9791189909420
|
딥러닝을 이용한 정형 데이터 분석
|
마크 라이언
|
책만
|
20220620
|
28,000
|
17
|
9791191600674
|
단단한 심층강화학습(I.A.I. 37)
|
로라 그레서
|
제이펍
|
20220217
|
30,000
|
18
|
9788965402282
|
인공지능을 위한 수학
|
이시카와 아키히코
|
프리렉
|
20181122
|
25,000
|
19
|
9791140700073
|
AI 상식사전
|
한규동
|
길벗
|
20220614
|
19,000
|
20
|
9791161756400
|
그래프 신경망 입문
|
즈위안 리우
|
에이콘출판
|
20220429
|
20,000
|
21
|
9791162245507
|
MLOps 도입 가이드
|
데이터이쿠
|
한빛미디어
|
20220429
|
22,000
|
22
|
9791165920395
|
선형대수와 통계학으로 배우는 머신러닝 with 파이썬
|
장철원
|
비제이퍼블릭
|
20210126
|
37,500
|
23
|
9791188621255
|
패턴 인식과 머신 러닝(제이펍의 인공지능 시리즈 11)
|
크리스토퍼 비숍
|
제이펍
|
20180910
|
46,000
|
24
|
9788956748337
|
파이썬 머신러닝 판다스 데이터 분석
|
오승환
|
정보문화사
|
20190615
|
25,000
|
25
|
9791162241974
|
김기현의 자연어 처리 딥러닝 캠프: 파이토치 편(소문난 명강의)
|
김기현
|
한빛미디어
|
20190701
|
38,000
|
26
|
9791162244975
|
비전 시스템을 위한 딥러닝
|
모하메드 엘겐디
|
한빛미디어
|
20211220
|
38,000
|
27
|
9791162245279
|
파이썬 라이브러리를 활용한 머신러닝(2판)
|
안드레아스 뮐러
|
한빛미디어
|
20220225
|
33,000
|
28
|
9791191905076
|
머신러닝·딥러닝 문제해결 전략(Must Have)
|
신백균
|
골든래빗(주)
|
20220413
|
38,000
|
29
|
9788931556872
|
엑셀로 배우는 머신러닝 초입문(AI의 얼개를 기본부터 설명한)
|
와쿠이 요시유키
|
성안당
|
20210222
|
23,000
|
30
|
9788954754071
|
수학과 함께하는 AI 기초(EBS)
|
EBS
|
EBS한국교육방송공사
|
20200908
|
12,000
|
31
|
9788970505350
|
AI, 빅데이터 활용이 쉬워지는 142가지 데이터셋
|
반병현
|
생능북스
|
20220222
|
22,000
|
32
|
9791161753508
|
한국어 임베딩
|
이기창
|
에이콘출판
|
20190926
|
35,000
|
33
|
9791162244845
|
머신러닝 디자인 패턴
|
발리아파 락슈마난
|
한빛미디어
|
20211101
|
38,000
|
34
|
9791162245392
|
XGBoost와 사이킷런을 활용한 그레이디언트 부스팅
|
코리 웨이드
|
한빛미디어
|
20220408
|
34,000
|
35
|
9791165215187
|
머신 러닝 교과서 with 파이썬, 사이킷런, 텐서플로(개정판 3판)
|
세바스찬 라시카
|
길벗
|
20210331
|
44,000
|
36
|
9791165215477
|
딥러닝 텐서플로 교과서
|
서지영
|
길벗
|
20210430
|
28,000
|
37
|
9791188621422
|
심층 학습(제이펍의 인공지능 시리즈 13)
|
이안 굿펠로
|
제이펍
|
20181031
|
42,000
|
38
|
9791189877880
|
노코딩 AI
|
하세정
|
예미
|
20220620
|
18,000
|
39
|
9788956748573
|
파이썬 딥러닝 파이토치(Python Deep Learning PyTorch)
|
이경택
|
정보문화사
|
20201008
|
25,000
|
40
|
9788960883932
|
비전공자를 위한 딥러닝(그림으로 이해하는)
|
윤준호
|
디지털북스
|
20220225
|
12,000
|
41
|
9788966262854
|
머신 러닝 딥 러닝에 필요한 기초 수학 with 파이썬(프로그래밍인사이트)
|
조준우
|
인사이트
|
20201104
|
27,000
|
42
|
9791158393052
|
실전! 파이토치 딥러닝 프로젝트(데이터 사이언스 시리즈 76)
|
아쉬쉬 란잔 자
|
위키북스
|
20220216
|
32,000
|
43
|
9791161756455
|
자연어 처리의 정석(데이터 과학)
|
제이콥 에이젠슈테인
|
에이콘출판
|
20220530
|
50,000
|
44
|
9791162243541
|
딥러닝 챗봇(처음 배우는)
|
조경래
|
한빛미디어
|
20201101
|
26,000
|
45
|
9791163031093
|
Do it! 정직하게 코딩하며 배우는 딥러닝 입문
|
박해선
|
이지스퍼블리싱
|
20190920
|
19,800
|
46
|
9791189057145
|
자연어처리 바이블(고려대학교 정보대학 교재시리즈)
|
임희석
|
휴먼싸이언스
|
20191230
|
35,000
|
47
|
9791190665179
|
단단한 강화학습(아이러브 인공지능 27)
|
리처드 서튼
|
제이펍
|
20200331
|
35,000
|
48
|
9788965402930
|
프로그래머를 위한 강화학습
|
멀티코어
|
프리렉
|
20210331
|
25,000
|
49
|
9791156641582
|
기계 학습
|
오일석
|
한빛아카데미
|
20171205
|
32,000
|
50
|
9791156644972
|
딥러닝을 위한 선형대수학
|
길버트 스트랭
|
한빛아카데미
|
20200805
|
38,000
|
51
|
9791156645085
|
파이썬으로 만드는 인공지능(IT@ COOKBOOK)
|
오일석
|
한빛아카데미
|
20210105
|
31,000
|
52
|
9791156724643
|
나는 오렌지로 데이터 분석한다
|
임진숙
|
씨마스
|
20211201
|
20,000
|
53
|
9791158391256
|
유니티 ML-Agents(따라 하면서 배우는)(위키북스 데이터 사이언스 시리즈 23)
|
마이클 랜햄
|
위키북스
|
20181121
|
22,000
|
54
|
9791158392598
|
강화학습/심층강화학습 특강(위키북스 데이터 사이언스 시리즈 71)
|
아들랑 드 폰테베
|
위키북스
|
20210617
|
27,000
|
55
|
9791158392673
|
헬로! 인공지능 생활코딩 머신러닝: 실습편 with 오렌지3(위키북스 헬로! 인공지능 시리즈 2)
|
이고잉
|
위키북스
|
20210716
|
15,000
|
56
|
9791158392888
|
머신러닝 시스템 디자인 패턴(AI 엔지니어를 위한)(위키북스 데이터 사이언스 시리즈 75)
|
시부이 유우스케
|
위키북스
|
20211124
|
32,000
|
57
|
9791161754420
|
생명과학을 위한 딥러닝(데이터 과학 시리즈)
|
바라스 람순다르
|
에이콘출판
|
20200819
|
25,000
|
58
|
9791161756165
|
디지털 트윈 개발 및 클라우드 배포
|
나심 칼레드
|
에이콘출판
|
20220225
|
45,000
|
59
|
9791162241080
|
미술관에 GAN 딥러닝 실전 프로젝트
|
데이비드 포스터
|
한빛미디어
|
20191115
|
32,000
|
60
|
9791162241387
|
기계는 어떻게 생각하고 학습하는가
|
뉴 사이언티스트
|
한빛미디어
|
20181205
|
17,000
|
61
|
9791162243435
|
GAN 인 액션
|
야쿠프 란그르
|
한빛미디어
|
20200917
|
30,000
|
62
|
9791162244333
|
파이토치로 배우는 자연어 처리
|
델립 라오
|
한빛미디어
|
20210601
|
26,000
|
63
|
9791162244913
|
AI로 일하는 기술
|
장동인
|
한빛미디어
|
20220103
|
18,000
|
64
|
9791162245415
|
케라스로 구현하는 딥러닝
|
김성진
|
한빛미디어
|
20220401
|
30,000
|
65
|
9791185553832
|
AI 인공지능 자율주행 자동차
|
장문철
|
앤써북
|
20210830
|
20,000
|
66
|
9791185553955
|
인공지능 자율주행 RC카 체험하고 코딩하기 with 라즈베리파이
|
서민우
|
앤써북
|
20220405
|
18,000
|
67
|
9791185553979
|
머신러닝 딥러닝을 이용한 A.I. 자율주행 RC카 프로젝트 with 파이썬+아두이노
|
서민우
|
앤써북
|
20220520
|
18,000
|
68
|
9791185578958
|
AI시대의 컴퓨터 개론
|
강환수
|
인피니티북스
|
20220217
|
29,000
|
69
|
9791188621989
|
단단한 머신러닝(아이러브 인공지능 24)
|
조우쯔화
|
제이펍
|
20200228
|
30,000
|
70
|
9791191600742
|
비전공자를 위한 인공지능 교과서(제이펍의 인공지능 시리즈 38)
|
후쿠마 도모키
|
제이펍
|
20220504
|
18,000
|
71
|
9788931463170
|
바닥부터 배우는 강화 학습
|
노승은
|
영진닷컴
|
20200915
|
22,000
|
72
|
9788931465914
|
딥러닝을 위한 파이토치 입문
|
딥러닝호형
|
영진닷컴
|
20220120
|
25,000
|
73
|
9788956749167
|
인공지능 바이블
|
조민호
|
정보문화사
|
20220630
|
28,000
|
74
|
9788958974062
|
모두를 위한 인공지능과 윤리
|
김성애
|
삼양미디어
|
20220705
|
23,000
|
75
|
9788970504728
|
처음 만나는 인공지능(개정판)
|
김대수
|
생능출판
|
20201209
|
26,000
|
76
|
9788970504872
|
쉬운 딥러닝
|
반병현
|
생능북스
|
20210421
|
20,000
|
77
|
9788970505237
|
인공지능이 비즈니스 모델이 되기까지
|
이지은
|
생능북스
|
20220103
|
21,000
|
78
|
9788970509594
|
인공지능
|
이건명
|
생능출판
|
20180903
|
35,000
|
79
|
9788980783113
|
OpenCV.Python 머신러닝 딥러닝 프로그래밍
|
김동근
|
가메
|
20220331
|
26,000
|
80
|
9791156009641
|
머신러닝 알고리즘 마스터(2판)
|
Giuseppe Bonaccorso
|
도서출판 홍릉(홍릉과학출판사)
|
20220331
|
55,000
|
81
|
9791156645504
|
난생처음 인공지능 입문(IT CookBook)
|
서지영
|
한빛아카데미
|
20210618
|
25,000
|
82
|
9791156724223
|
모두를 위한 인공지능 언플러그드
|
임진숙
|
씨마스
|
20210122
|
18,000
|
83
|
9791158391713
|
케라스로 구현하는 고급 딥러닝 알고리즘(위키북스 데이터 사이언스 시리즈 40)
|
로웰 아티엔자
|
위키북스
|
20190927
|
28,000
|
84
|
9791158391799
|
파이썬을 이용한 머신러닝, 딥러닝 실전 개발 입문(개정판)(위키북스 데이터 사이언스 시리즈 45)
|
쿠지라 히코우즈쿠에
|
위키북스
|
20191210
|
30,000
|
85
|
9791158392123
|
실전! 텐서플로 2를 활용한 딥러닝 컴퓨터 비전(위키북스 데이터 사이언스 시리즈 57)
|
벤자민 플랜치
|
위키북스
|
20200602
|
28,000
|
86
|
9791158392185
|
나의 첫 머신러닝/딥러닝(위키북스 데이터 사이언스 시리즈 60)
|
허민석
|
위키북스
|
20200730
|
26,000
|
87
|
9791158392666
|
헬로! 인공지능 생활코딩 머신러닝: 이론편(위키북스 헬로! 인공지능 시리즈 1)
|
이고잉
|
위키북스
|
20210716
|
13,000
|
88
|
9791160507652
|
OpenCV 4로 배우는 컴퓨터 비전과 머신 러닝
|
황선규
|
길벗
|
20190412
|
42,000
|
89
|
9791161750347
|
C++와 CUDA C로 구현하는 딥러닝 알고리즘 세트(에이콘 데이터 과학 시리즈)(전3권)
|
티모시 마스터즈
|
에이콘출판
|
20170818
|
78,000
|
90
|
9791161752334
|
실전 금융 머신러닝 완벽 분석(에이콘 금융 퀀트 머신러닝 융합 시리즈)
|
마르코스 로페즈 데 프라도
|
에이콘출판
|
20190102
|
40,000
|
91
|
9791161752426
|
피처 엔지니어링, 제대로 시작하기
|
앨리스 젱
|
에이콘출판
|
20190102
|
25,000
|
92
|
9791161753928
|
R을 활용한 머신러닝(3판)
|
브레트 란츠
|
에이콘출판
|
20200227
|
36,000
|
93
|
9791161754208
|
적대적 머신러닝(데이터 과학)
|
앤서니 조셉
|
에이콘출판
|
20200630
|
40,000
|
94
|
9791161754628
|
OpenCV 4를 활용한 머신러닝 입문(2판)(데이터 과학)
|
아디타 샤르마
|
에이콘출판
|
20201030
|
40,000
|
95
|
9791161755250
|
자율주행차량 기술 입문(임베디드 시스템)
|
행키 샤프리
|
에이콘출판
|
20210521
|
33,000
|
96
|
9791161755939
|
금융 머신러닝
|
매튜 딕슨
|
에이콘출판
|
20220111
|
60,000
|
97
|
9791161756134
|
MLFlow를 활용한 MLOps
|
스리다르 알라
|
에이콘출판
|
20220228
|
30,000
|
98
|
9791162241950
|
파이썬을 활용한 머신러닝 쿡북
|
크리스 알본
|
한빛미디어
|
20190901
|
33,000
|
99
|
9791162242278
|
펭귄브로의 3분 딥러닝 파이토치맛
|
김건우
|
한빛미디어
|
20191101
|
28,000
|
100
|
9791162243190
|
핸즈온 비지도 학습
|
안쿠르 A. 파텔
|
한빛미디어
|
20200720
|
34,000
|
101
|
9791162244234
|
데이터가 뛰어노는 AI 놀이터, 캐글
|
가도와키 다이스케
|
한빛미디어
|
20210510
|
35,000
|
102
|
9791162244692
|
머신러닝 파워드 애플리케이션
|
에마뉘엘 아메장
|
한빛미디어
|
20210906
|
27,000
|
103
|
9791162244814
|
살아 움직이는 머신러닝 파이프라인 설계
|
하네스 하프케
|
한빛미디어
|
20211011
|
32,000
|
104
|
9791162244838
|
그로킹 심층 강화학습
|
미겔 모랄레스
|
한빛미디어
|
20211010
|
35,000
|
105
|
9791162244876
|
머신러닝&딥러닝 with 파이썬(한 권으로 다지는)
|
알베르토 아르타산체스
|
한빛미디어
|
20211021
|
40,000
|
106
|
9791162245002
|
금융 전략을 위한 머신러닝
|
하리옴 탓샛
|
한빛미디어
|
20211227
|
35,000
|
107
|
9791162245521
|
쉽게 배우는 AWS AI 서비스
|
피터 엘거
|
한빛미디어
|
20220415
|
30,000
|
108
|
9791162263877
|
비즈니스를 위한 AI(2판)
|
Doug Rose
|
시그마프레스
|
20220630
|
23,000
|
109
|
9791163031253
|
기계는 어떻게 생각하는가?
|
숀 게리시
|
이지스퍼블리싱
|
20191227
|
18,000
|
110
|
9791163033431
|
Do it! 첫 인공지능
|
이애리
|
이지스퍼블리싱
|
20220401
|
13,000
|
111
|
9791165219239
|
구글 브레인 팀에게 배우는 딥러닝 with TensorFlow.js
|
샨칭 차이
|
길벗
|
20220331
|
44,000
|
112
|
9791165921125
|
알고리즘 구현으로 배우는 선형대수 with 파이썬
|
장철원
|
비제이퍼블릭
|
20211224
|
34,000
|
113
|
9791185459547
|
마스터 알고리즘
|
페드로 도밍고스
|
비즈니스북스
|
20160730
|
22,000
|
114
|
9791188621804
|
알파제로를 분석하며 배우는 인공지능(제이펍의 인공지능 시리즈 22)
|
후루카와 히데카즈
|
제이펍
|
20191230
|
30,000
|
115
|
9791189909024
|
실체가 손에 잡히는 딥러닝, 기초부터 실전 프로그래밍
|
아즈마 유키나가
|
책만
|
20190618
|
26,000
|
116
|
9791190665230
|
데이터 과학자와 데이터 엔지니어를 위한 인터뷰 문답집(아이러브 인공지능 28)
|
Hulu 데이터 과학팀
|
제이펍
|
20200630
|
34,000
|
117
|
9791196965624
|
클라우드 모바일 및 에지 기반의 딥러닝 실용가이드
|
Anirudh Koul
|
DK로드북스
|
20200707
|
42,000
|
118
|
9791197249006
|
알파(R과 Python)를 활용한 인공지능 챗봇 14
|
황윤찬
|
STORYJOA(스토리조아)
|
20201230
|
23,000
|
119
|
9788909546676
|
코딩스쿨 엔트리로 시작하는 인공지능 프로그래밍
|
정인기
|
교학사
|
20211020
|
9,000
|
120
|
9788931456639
|
구글에서 배우는 딥러닝
|
닛케이 빅데이터
|
영진닷컴
|
20170905
|
16,000
|
121
|
9788931555660
|
C++로 배우는 딥러닝(신경망의 기초부터 C++를 이용한 구현까지)
|
후지타 타케시
|
성안당
|
20180629
|
23,000
|
122
|
9788931556704
|
엑셀로 배우는 딥러닝
|
와쿠이 요시유키
|
성안당
|
20200525
|
23,000
|
123
|
9788931556766
|
엑셀로 배우는 순환 신경망· 강화학습 초입문: RNN(순환신경망).DQN(심층Q-네트워크)편
|
와쿠이 요시유키
|
성안당
|
20200724
|
23,000
|
124
|
9788931556926
|
한눈에 보이는 인공지능 그림책
|
한선관
|
성안당
|
20210422
|
19,000
|
125
|
9788931588057
|
머신러닝(만화로 쉽게 배우는)
|
아라키 마사히로
|
성안당
|
20190522
|
15,000
|
126
|
9788956749006
|
파이썬 딥러닝 머신러닝 입문
|
오승환
|
정보문화사
|
20210105
|
25,000
|
127
|
9788958207429
|
코딩책과 함께 보는 인공지능 개념 사전
|
김현정
|
궁리
|
20211005
|
16,000
|
128
|
9788960777491
|
머신 러닝(Machine Learning)(에이콘 데이터 과학 시리즈)
|
케빈 머피
|
에이콘출판
|
20150831
|
60,000
|
129
|
9788960778580
|
C++와 CUDA C로 구현하는 딥러닝 알고리즘 1(에이콘 데이터과학 시리즈)
|
티모시 마스터즈
|
에이콘출판
|
20160428
|
30,000
|
130
|
9788960779662
|
자바 딥러닝의 핵심(acorn+PACKT 시리즈)
|
유스케 스고모리
|
에이콘출판
|
20170125
|
25,000
|
131
|
9788968483509
|
신경망 첫걸음(첫걸음 시리즈)
|
타리크 라시드
|
한빛미디어
|
20170403
|
25,000
|
132
|
9788970505121
|
실습하며 배우는 딥러닝 입문 with Kaggle
|
장원두
|
생능출판
|
20210730
|
29,000
|
133
|
9788981720353
|
기초퍼지이론
|
유동선
|
교우사
|
19980302
|
25,000
|
134
|
9788981720544
|
퍼지과학의 세계
|
장이채
|
교우사
|
19970301
|
11,000
|
135
|
9788997924387
|
1억배 빠른 양자 컴퓨터가 온다(누구나 읽을 수 있는 IT 과학이야기 3)
|
니시모리 히데토시
|
로드북
|
20180416
|
15,000
|
136
|
9791125102373
|
인공지능 입문(수학이론과 실습)(너도 나도 따라할 수 있는)
|
민조홍
|
교우
|
20180228
|
15,000
|
137
|
9791156008699
|
머신러닝 데이터 분석(오렌지3로 알아가는)
|
손원성
|
홍릉
|
20210805
|
39,000
|
138
|
9791158391393
|
실전! 딥러닝(데이터 사이언스 시리즈 29)
|
오타 미쯔히사
|
위키북스
|
20190215
|
25,000
|
139
|
9791158392000
|
XAI 설명 가능한 인공지능, 인공지능을 해부하다(위키북스 데이터 사이언스 시리즈 53)
|
안재현
|
위키북스
|
20200327
|
28,000
|
140
|
9791158392321
|
금융 전문가를 위한 머신러닝 알고리즘(위키북스 데이터 사이언스 시리즈 65)
|
얀네스 클라스
|
위키북스
|
20210107
|
35,000
|
141
|
9791161751726
|
파이썬 자연어 처리의 이론과 실제
|
잘라지 트하나키
|
에이콘출판
|
20180629
|
35,000
|
142
|
9791161752594
|
PyTorch로 시작하는 딥러닝
|
비슈누 수브라마니안
|
에이콘출판
|
20190218
|
25,000
|
143
|
9791161752761
|
텐서플로를 이용한 고급 딥러닝(데이터 과학 시리즈)
|
산타누 파타나야크
|
에이콘출판
|
20190228
|
35,000
|
144
|
9791161753409
|
The Hundred-Page Machine Learning Book(데이터 과학)
|
안드리 부르코프
|
에이콘출판
|
20190830
|
25,000
|
145
|
9791161753782
|
케라스로 배우는 신경망 설계와 구현
|
닐로이 푸르카이트
|
에이콘출판
|
20200221
|
40,000
|
146
|
9791161754109
|
텐서플로 2와 케라스로 구현하는 딥러닝(2판)(데이터 과학)
|
안토니오 걸리
|
에이콘출판
|
20200429
|
43,000
|
147
|
9791161754918
|
자산운용을 위한 금융 머신러닝(금융 퀀트 머신러닝 융합 시리즈)
|
마르코스 로페즈 데 프라도
|
에이콘출판
|
20210126
|
20,000
|
148
|
9791161755878
|
추천 시스템
|
차루 아가르왈
|
에이콘출판
|
20211231
|
40,000
|
149
|
9791161756448
|
그림으로 배우는 딥러닝
|
앤드류 글래스너
|
에이콘출판
|
20220531
|
60,000
|
150
|
9791161756462
|
추천 시스템의 통계 기법(데이터 과학)
|
디팍 아가왈
|
에이콘출판
|
20220531
|
33,000
|
151
|
9791162240045
|
처음 배우는 머신러닝
|
김승연
|
한빛미디어
|
20171001
|
26,000
|
152
|
9791162240441
|
처음 배우는 딥러닝 수학
|
와쿠이 요시유키
|
한빛미디어
|
20180201
|
25,000
|
153
|
9791162241073
|
텐서플로를 활용한 머신러닝
|
니샨트 수클라
|
한빛미디어
|
20190201
|
30,000
|
154
|
9791162241899
|
파이토치 첫걸음
|
최건호
|
한빛미디어
|
20190607
|
23,000
|
155
|
9791162242001
|
파이썬 날코딩으로 알고 짜는 딥러닝
|
윤덕호
|
한빛미디어
|
20190715
|
45,000
|
156
|
9791162243312
|
퀀트 전략을 위한 인공지능 트레이딩
|
김태헌
|
한빛미디어
|
20200820
|
28,000
|
157
|
9791162243411
|
초소형 머신러닝 TinyML
|
피트 워든
|
한빛미디어
|
20200820
|
40,000
|
158
|
9791162243954
|
GAN 첫걸음
|
타리크 라시드
|
한빛미디어
|
20210310
|
25,000
|
159
|
9791162244357
|
머신러닝을 활용한 웹 최적화
|
이쓰카 슈헤이
|
한빛미디어
|
20210607
|
34,000
|
160
|
9791162244593
|
텐서플로 라이트를 활용한 안드로이드 딥러닝
|
임태규
|
한빛미디어
|
20210720
|
27,000
|
161
|
9791162244609
|
만들면서 배우는 파이토치 딥러닝
|
오가와 유타로
|
한빛미디어
|
20210805
|
40,000
|
162
|
9791162244630
|
fastai와 파이토치가 만나 꽃피운 딥러닝
|
제러미 하워드
|
한빛미디어
|
20210810
|
44,000
|
163
|
9791162244647
|
웹 브라우저 속 머신러닝 TensorFlow.js
|
카이 사사키
|
한빛미디어
|
20210901
|
25,000
|
164
|
9791162245323
|
머신러닝 실무 프로젝트(2판)
|
아리가 미치아키
|
한빛미디어
|
20220310
|
24,000
|
165
|
9791163032991
|
Do it! 딥러닝 교과서
|
윤성진
|
이지스퍼블리싱
|
20211018
|
28,000
|
166
|
9791165213985
|
모두의 인공지능 with 파이썬
|
이영호
|
길벗
|
20201230
|
22,000
|
167
|
9791165216726
|
쉽게 시작하는 캐글 데이터 분석
|
시노다 히로유키
|
길벗
|
20210908
|
22,000
|
168
|
9791165920753
|
파이토치 딥러닝 프로젝트 모음집(한 줄씩 따라 해보는)
|
이경택
|
비제이퍼블릭
|
20210730
|
29,000
|
169
|
9791185578606
|
인공지능
|
천인국
|
인피니티북스
|
20200212
|
30,000
|
170
|
9791185890944
|
그림과 수식으로 배우는 통통 인공지능(제이펍의 인공지능 시리즈 8)
|
타니구치 타다히로
|
제이펍
|
20170929
|
23,000
|
171
|
9791187512592
|
세상에서 가장 쉬운 AI 앱 수업
|
공민수
|
리틀에이
|
20210930
|
17,500
|
172
|
9791188508198
|
엑사스케일과 인공지능 그리고 양자컴퓨터(지금부터 시작하는)
|
추형석
|
위즈플래닛
|
20211120
|
15,000
|
173
|
9791188621774
|
파이썬으로 배우는 응용 텍스트 분석(제이펍의 인공지능 시리즈 20)
|
벤자민 벵포트
|
제이펍
|
20191129
|
27,000
|
174
|
9791189057220
|
인공지능 베이직
|
Tom Taulli
|
휴먼싸이언스
|
20201020
|
19,000
|
175
|
9791189184032
|
딥러닝을 위한 최적화와 수치해석
|
황윤구
|
남가람북스
|
20200203
|
32,000
|
176
|
9791189909031
|
실무가 훤히 보이는 머신러닝 & 딥러닝
|
마창수
|
책만
|
20190731
|
26,000
|
177
|
9791189909246
|
핵심 딥러닝 입문: RNN, LSTM, GRU, VAE, GAN 구현
|
아즈마 유키나가
|
책만
|
20201209
|
28,000
|
178
|
9791190014823
|
당신이 지금 알아야 할 빅4 클라우드 머신러닝
|
이영호
|
비제이퍼블릭
|
20200331
|
26,000
|
179
|
9791191346350
|
인공지능 코딩하기(허스키렌즈와 마이크로비트로 배우는)
|
이수진
|
지오북스
|
20220223
|
19,000
|
180
|
9791191600322
|
인공지능 2(4판)(제이펍의 인공지능 시리즈 33)
|
스튜어트 러셀
|
제이펍
|
20210825
|
38,000
|
181
|
9791195484584
|
Gpu의 모든 기술(머신러닝과 블록체인을 떠받치는)
|
Hisa Ando
|
남가람북스
|
20180414
|
30,000
|
182
|
9791196262044
|
만화로 이해하는 인공지능
|
칸 요오코 (그림)
|
보국출판사
|
20210216
|
15,000
|
183
|
9791197175923
|
할로코드로 배우는 인공지능 코딩 활용편
|
최만
|
슈퍼트랙
|
20210915
|
18,000
|
184
|
9791197420603
|
딥러닝 개념과 활용(양장본 HardCover)
|
김의중
|
미리어드스페이스
|
20210416
|
35,000
|
교보문고 컴퓨터공학분야 국내도서 베스트셀러 최근1주일 기준 (2022년 7월 13일) (0) | 2022.07.13 |
---|---|
Amazon에서 MXNet 기반으로 작성한 유명한 텍스트북 Dive Into Deep Learning 에 PyTorch 버전의 코드들이 수록된 (0) | 2020.07.08 |
9 Best AI & Machine Learning Books To Read In 2020 (0) | 2020.04.24 |
한빛미디어 AI 서적들 (0) | 2020.01.08 |
교보문고 2019 IT 전문서 올해의 책 (0) | 2020.01.08 |
windows 10 파이참pycharm 이용 시 주석처리 단축키는 'Ctrl + /'인데,
이 단축키가 갑자기 작동이 안되고 '/'가 입력됐다.
검색해보니 https://dev-jinee.tistory.com/3 에서는 'Ctrl + Shift' 를 눌러 Microsoft 입력기로 바꿔주면 된다고 했는데,
나는 '윈도우키 + 스페이스'를 눌러 바꿔줄 수 있었다.
1. 파이참Pycharm으로 원격 서버 연결해서 코드 수정하려면 일단 원격서버에 SSH를 설치해야한다.
https://lucidmaj7.tistory.com/178 를 참조해서 설치하자
2. 그 후 아래 사이트들을 참조하여 파이참 프로페셔널 버전으로 연결하여 수정할 수 있다.
https://wisdomcoder.oopy.io/6358c13a-0b75-42b5-adf9-e31f17b5901b , https://epicarts.tistory.com/99
https://www.kaggle.com/willkoehrsen/automated-feature-engineering-basics/notebook
https://medium.com/analytics-vidhya/feature-engineering-using-featuretools-with-code-10f8c83e5f68 (https://github.com/ranasingh-gkp/Feature_engineering_Featuretools)
https://data-newbie.tistory.com/815 은 원본코드 https://www.kaggle.com/frednavruzov/auto-feature-generation-featuretools-example 를 버전 업데이트에 맞춰 수정한 최신 코드이다.
Featuretools의 공식 홈페이지의 예제코드
https://featuretools.alteryx.com/en/stable/ , https://featuretools.alteryx.com/en/stable/getting_started/using_entitysets.html
https://youtube.com/playlist?list=PLSlDi2AkDv832sRHreZKoyyzJnGxLkcfF
Feature Tools에 대한 설문 문서 페이지들: https://primitives.featurelabs.com/ , https://docs.featuretools.com/en/stable/api_reference.html#feature-primitives
* 아래는 PCA를 이용한 Feature Extraction 설명 및 코드
https://vitalflux.com/feature-extraction-pca-python-example/
참고원문: https://www.activestate.com/resources/quick-reads/how-to-update-all-python-packages/
# https://www.activestate.com/resources/quick-reads/how-to-update-all-python-packages/
import pkg_resources
from subprocess import call
for dist in pkg_resources.working_set:
call("python -m pip install --upgrade " + dist.project_name, shell=True)
원문의 dist.<projectname> --> dist.project_name 로 수정
[python] [ipynb to py 변환 방법] jupyter nbconvert --to python [filename].ipynb (0) | 2021.04.27 |
---|---|
python 주피터 노트북(jupyter notebook) 색상 및 테마변경하기 (0) | 2020.11.25 |
How can we improve coding skill?? (0) | 2020.11.20 |
Python youtube_dl.YoutubeDL() Examples (0) | 2020.11.03 |
youtube-dl옵션 자세히 설명 (youtube-dl - download videos from youtube.com or other video platforms) (0) | 2020.11.03 |
https://bestpractice80.tistory.com/57
SSL 통시늘 시도 시 회사에서는 방화벽 문제로 인해서 Server Certificates 오류가 발생 할 수 있다.
* 해결 방법
- SSL 통신 옵션 해제
File > Settings > Tools > Server Certificates > Accept non-trusted certificates automatically 체크
File > Settings > Appearance & Behavior > System Settings > Updates > Use Secure connection 체크 해제
참고자료 :
https://www.jetbrains.com/help/idea/server-certificates.html
https://intellij-support.jetbrains.com/hc/en-us/community/posts/206773929-sun-security-validator-ValidatorException-when-trying-to-use-CLion-Updater
https://www.jetbrains.com/help/hub/Trusted-SSL-Certificates.html
windows 10 파이참pycharm 이용 시 주석처리 단축키 'Ctrl + /'작동 안될 때 (0) | 2022.07.13 |
---|---|
파이참Pycharm으로 원격 서버 SSH 연결해서 코드 수정하기 (윈도우) (0) | 2022.02.25 |
[pycharm파이참에서 import 자동으로 hide숨김을 해제하는 방법]How do I make pyCharm stop hiding (unfold) my Python imports? (0) | 2021.11.22 |
파이참pycharm에서 2개 이상의 multiple projects 돌리는 방법 (0) | 2021.03.06 |
[self] pycharm에서 graphviz를 conda로 설치하니 안되는데, pip로 설치하니 된다. (0) | 2020.12.16 |
As this question may be useful for people who also are not looking for the term "code folding", I'll make my comment an answer.
As extracted from IntelliJ IDE Web Help, but also worked on PyCharm CE 3.4.1:
The image below shows what it looks like:
----------------------------------------------------
Updated
File -> Setting -> Editor -> General -> Code Folding -> at 'Fold by default', uncheck 'Imports'
파이참Pycharm으로 원격 서버 SSH 연결해서 코드 수정하기 (윈도우) (0) | 2022.02.25 |
---|---|
pycharm untrusted server certificate popup [파이참] Server Certificates 오류 해결 (0) | 2021.11.25 |
파이참pycharm에서 2개 이상의 multiple projects 돌리는 방법 (0) | 2021.03.06 |
[self] pycharm에서 graphviz를 conda로 설치하니 안되는데, pip로 설치하니 된다. (0) | 2020.12.16 |
virtual env을 pycharm에 연동 시키는 방법 (0) | 2019.04.03 |
https://stackoverflow.com/questions/48520524/how-to-debug-code-running-from-terminal-in-pycharm
2가지 방법이 있다.
[1번 방법] : 위 링크의 1번째 나오는 방법
I don't know anyway to do this through the terminal, but the way I currently do it is:
With the right configuration the result should be the same as running the script in a terminal.
I find the above process a little tedious. A PEP 553 breakpoint() might be nicer. It requires Python 3.7 and is not implemented yet. See the related issue.
[2번 방법] : 위 링크의 2번째 나오는 방법. 아래설명에서처럼 Tools -> Python or Debug Console로 IDE밑에 터미널이 나오면, 아래 그림에서처럼 Attach Debugger 버튼 누르고 breakpoint 건다. 그 후 '%run main.py' 이런 식으로 실행시키면 breakpoint에 디버깅이 걸린다.
ex) command window에서 'python code/__init__.py --img1 images/aligned_images/suji1_01.png --img2 images/aligned_images/suji2_01.png --output output3.mp4' 라고 실행했다면,
'%run code/__init__.py --img1 images/aligned_images/suji1_01.png --img2 images/aligned_images/suji2_01.png --output output3.mp4'라고 IDE 밑 터미널 창에 입력해준다.
There is a way using python console.
To run it: Tools -> python console .. or you can find it at the bottom of the IDE.
Then press on 'Attach debugger' line in the following picture:
Add a breakpoint in your code and then pass the file name to the cosole, like: %run my_file.py
위 사이트에 accuracy, F1, precision and recall만 구하는데, 내가 iou 구하는 코드도 추가했다.
def iou_m(y_true, y_pred, dtype=tf.float32):
# tf tensor casting
y_pred = tf.convert_to_tensor(y_pred)
y_pred = tf.cast(y_pred, dtype)
y_true = tf.cast(y_true, y_pred.dtype)
y_pred = tf.squeeze(y_pred)
y_true = tf.squeeze(y_true)
y_true_pos = tf.reshape(y_true, [-1])
y_pred_pos = tf.reshape(y_pred, [-1])
area_intersect = tf.reduce_sum(tf.multiply(y_true_pos, y_pred_pos))
area_true = tf.reduce_sum(y_true_pos)
area_pred = tf.reduce_sum(y_pred_pos)
area_union = area_true + area_pred - area_intersect
return tf.math.divide_no_nan(area_intersect, area_union)
-------------------------------------------------------------------------------------------
from keras import backend as K
def recall_m(y_true, y_pred):
true_positives = K.sum(K.round(K.clip(y_true * y_pred, 0, 1)))
possible_positives = K.sum(K.round(K.clip(y_true, 0, 1)))
recall = true_positives / (possible_positives + K.epsilon())
return recall
def precision_m(y_true, y_pred):
true_positives = K.sum(K.round(K.clip(y_true * y_pred, 0, 1)))
predicted_positives = K.sum(K.round(K.clip(y_pred, 0, 1)))
precision = true_positives / (predicted_positives + K.epsilon())
return precision
def f1_m(y_true, y_pred):
precision = precision_m(y_true, y_pred)
recall = recall_m(y_true, y_pred)
return 2*((precision*recall)/(precision+recall+K.epsilon()))
def iou_m(y_true, y_pred, dtype=tf.float32):
# tf tensor casting
y_pred = tf.convert_to_tensor(y_pred)
y_pred = tf.cast(y_pred, dtype)
y_true = tf.cast(y_true, y_pred.dtype)
y_pred = tf.squeeze(y_pred)
y_true = tf.squeeze(y_true)
y_true_pos = tf.reshape(y_true, [-1])
y_pred_pos = tf.reshape(y_pred, [-1])
area_intersect = tf.reduce_sum(tf.multiply(y_true_pos, y_pred_pos))
area_true = tf.reduce_sum(y_true_pos)
area_pred = tf.reduce_sum(y_pred_pos)
area_union = area_true + area_pred - area_intersect
return tf.math.divide_no_nan(area_intersect, area_union)
# compile the model
model.compile(optimizer='adam', loss='binary_crossentropy', metrics=['acc',f1_m,precision_m, recall_m])
# fit the model
history = model.fit(Xtrain, ytrain, validation_split=0.3, epochs=10, verbose=0)
# evaluate the model
loss, accuracy, f1_score, precision, recall = model.evaluate(Xtest, ytest, verbose=0)
2020년 가을에 UMASS에서 개설된 Advanced NLP 강의입니다.
슬라이드/동영상 모두 제공됩니다.
강의 제목처럼 기본 NLP내용 외에 최신 내용들을 다루기 때문에 NLP에 대한 사전 지식이 필요한 강의입니다.
동영상 강의는 총 26시간 정도 분량입니다.
[video] https://www.youtube.com/playlist?list=PLWnsVgP6CzadmQX6qevbar3_vDBioWHJL
[homepage] https://people.cs.umass.edu/~miyyer/cs685/schedule.html
[schedule]
Week 1: introduction, language models, representation learning
Week 2: neural LMs, RNNs, backpropagation
Week 3: Attention mechanisms
Week 4: Transformers, transfer learning
Week 5: BERT and how to use it for downstream tasks
Week 6: further improving transfer learning in NLP
Week 7: improving text generation
Week 8: data augmentation and collection
Week 9: model distillation and retrieval-augmented LMs
Week 10: Transformer implementation, vision + language
Week 11: Exam week!
Week 12: Ethics and probe tasks
Week 13: Semantic parsing and commonsense reasoning
이미 많은 분들이 아실 것 같지만, AI에 필요한 수학 관련한 좋은 무료 강좌들입니다.
특히 3Blue1Brown과 Seeing Theory 컨텐츠는 워낙 쉽게 잘 설명을 해놓았고, visulization이 좋아서 입문자에게 좋습니다.
1. Linear Algebra
- 3Blue1Brown 채널 : https://www.youtube.com/playlist?list=PLZHQObOWTQDPD3MizzM2xVFitgF8hE_ab
- MIT 강의 : https://ocw.mit.edu/courses/mathematics/18-06-linear-algebra-spring-2010/video-lectures/
2. Probability
- Harvard 강의 : https://www.youtube.com/playlist?list=PL2SOU6wwxB0uwwH80KTQ6ht66KWxbzTIo
- Seeing Theory : https://seeing-theory.brown.edu/index.html#firstPage
3. Calculus
- 3Blue1Brown 채널 : https://www.youtube.com/playlist?list=PLZHQObOWTQDMsr9K-rj53DwVRMYO3t5Yr
4. Multivarate Calculus
- Coursera 강의 : https://www.coursera.org/learn/multivariate-calculus-machine-learning
Originally from Santiago's tweet
jupyter nbconvert --to script [filename].ipynb
or
jupyter nbconvert --to python [filename].ipynb
파이썬 모든 패키지,라이브러리 업데이트 하기,Updating All Packages In A Virtual Environment on Windows (0) | 2022.02.18 |
---|---|
python 주피터 노트북(jupyter notebook) 색상 및 테마변경하기 (0) | 2020.11.25 |
How can we improve coding skill?? (0) | 2020.11.20 |
Python youtube_dl.YoutubeDL() Examples (0) | 2020.11.03 |
youtube-dl옵션 자세히 설명 (youtube-dl - download videos from youtube.com or other video platforms) (0) | 2020.11.03 |
결론은 summation across chaannel이다. 각 채널별로 콘볼루션 계산 후 더하여 output을 만든다.
[1] https://stackoverflow.com/questions/43306323/keras-conv2d-and-input-channels
[2] https://datascience.stackexchange.com/questions/76456/why-do-we-use-2d-kernel-for-rgb-data
----------------------------------------------------------------------------------------------------------------
The Keras layer documentation specifies the input and output sizes for convolutional layers: https://keras.io/layers/convolutional/
Input shape: (samples, channels, rows, cols)
Output shape: (samples, filters, new_rows, new_cols)
And the kernel size is a spatial parameter, i.e. detemines only width and height.
So an input with c channels will yield an output with filters channels regardless of the value of c. It must therefore apply 2D convolution with a spatial height x width filter and then aggregate the results somehow for each learned filter.
What is this aggregation operator? is it a summation across channels? can I control it? I couldn't find any information on the Keras documentation.
Thanks.
Follow
asked Apr 9 '17 at 11:46
1,5584 gold badges15 silver badges24 bronze badges
38
It might be confusing that it is called Conv2D layer (it was to me, which is why I came looking for this answer), because as Nilesh Birari commented:
I guess you are missing it's 3D kernel [width, height, depth]. So the result is summation across channels.
Perhaps the 2D stems from the fact that the kernel only slides along two dimensions, the third dimension is fixed and determined by the number of input channels (the input depth).
For a more elaborate explanation, read https://petewarden.com/2015/04/20/why-gemm-is-at-the-heart-of-deep-learning/
I plucked an illustrative image from there:
Follow
answered Jul 12 '17 at 10:26
5,4357 gold badges37 silver badges61 bronze badges
Report this ad
34
I was also wondering this, and found another answer here, where it is stated (emphasis mine):
Maybe the most tangible example of a multi-channel input is when you have a color image which has 3 RGB channels. Let's get it to a convolution layer with 3 input channels and 1 output channel. (...) What it does is that it calculates the convolution of each filter with its corresponding input channel (...). The stride of all channels are the same, so they output matrices with the same size. Now, it sums up all matrices and output a single matrix which is the only channel at the output of the convolution layer.
Illustration:
Notice that the weights of the convolution kernels for each channel are different, which are then iteratively adjusted in the back-propagation steps by e.g. gradient decent based algorithms such as stochastic gradient descent (SDG).
Here is a more technical answer from TensorFlow API.
Follow
answered May 13 '18 at 14:36
1,52511 silver badges9 bronze badges
28
I also needed to convince myself so I ran a simple example with a 3×3 RGB image.
# red # green # blue 1 1 1 100 100 100 10000 10000 10000 1 1 1 100 100 100 10000 10000 10000 1 1 1 100 100 100 10000 10000 10000
The filter is initialised to ones:
1 1 1 1
I have also set the convolution to have these properties:
We would expect the (aggregated) output to be:
40404 40404 40404 40404
Also, from the picture above, the no. of parameters is
3 separate filters (one for each channel) × 4 weights + 1 (bias, not shown) = 13 parameters
Here's the code.
Import modules:
import numpy as np from keras.layers import Input, Conv2D from keras.models import Model
Create the red, green and blue channels:
red = np.array([1]*9).reshape((3,3)) green = np.array([100]*9).reshape((3,3)) blue = np.array([10000]*9).reshape((3,3))
Stack the channels to form an RGB image:
img = np.stack([red, green, blue], axis=-1) img = np.expand_dims(img, axis=0)
Create a model that just does a Conv2D convolution:
inputs = Input((3,3,3)) conv = Conv2D(filters=1, strides=1, padding='valid', activation='relu', kernel_size=2, kernel_initializer='ones', bias_initializer='zeros', )(inputs) model = Model(inputs,conv)
Input the image in the model:
model.predict(img) # array([[[[40404.], # [40404.]], # [[40404.], # [40404.]]]], dtype=float32)
Run a summary to get the number of params:
model.summary()
Follow
answered Dec 26 '18 at 9:37
1,43717 silver badges22 bronze badges
[2] https://datascience.stackexchange.com/questions/76456/why-do-we-use-2d-kernel-for-rgb-data
I have recently started kearning CNN and I coukdnt understand that why are we using a 2D kernel like of shape (3x3) for a RGB data in place of a 3D kernel like of shape (3x3x3)?
Are we sharing the same kernel among all the channels because the data would look the same in all the channels?
-->
welcome to the community
I guess there's a confusion in your understanding of the kernel we use in case of rgb data. We normally use a kernel of equal number of channels as the input coming in (in this case as you mentioned it's RGB, so my number of channels for convolution operation would be 3). So instead of using a 3 X 3 Kernel, we use a 3 X 3 X 3 kernel. Weight matrix multiplication of kernel and image pixels happen channel-wise.
However, having said this, you can use a kernel of size 3 X 3 when input image is rgb, by specifying the stride as 1 in the third dimension. What this will do is convolute the kernel not only horizontally and vertically but also through the depth or specifically through the channels as well. I don't exactly know why one would like to do it.
Apart from this I guess the course or the video you are referring to might have specified '2D convolution on a 3D image'. That doesn't mean using a 2D kernel. And a 2D convolution on a 3D image uses a 3D kernel and after weight matrix multiplication you get a 2D image hence justifying the 2D convolution name.
[arxiv.org에 올라온 논문을 pdf가 아닌 잘 정돈된 1 column의 텍스트 페이지로 볼 수 있는 방법] 주소 창에서 arxiv의 x를 숫자 5로 바꾸면 pdf가 아닌 텍스트 페이지로 나옴 (0) | 2022.10.17 |
---|---|
NVIDIA Research Unveils Flowtron, an Expressive and Natural Speech Synthesis Mod (0) | 2020.05.15 |
수학을 포기한 직업 프로그래머가 머신러닝 학습을 시작하기위한 학습법 소개 (0) | 2017.07.27 |
파이썬 쥬피터를 이용한 텐서플로우 개발환경 구성하기 (0) | 2017.04.13 |
Why Momentum Really Works (0) | 2017.04.05 |
윈도우 10에 텐서플로우 2.x버전에서 gpu로 실행 시
failed to create cublas handle: CUBLAS_STATUS_ALLOC_FAILED 에러 날 때는
import tensorflow as tf
config = tf.compat.v1.ConfigProto(gpu_options = tf.compat.v1.GPUOptions(per_process_gpu_memory_fraction=0.8)
#device_count = {'GPU': 1}
)
config.gpu_options.allow_growth = True
session = tf.compat.v1.Session(config=config)
tf.compat.v1.keras.backend.set_session(session)
를 코드 상단에 추가해주자.
github.com/tensorflow/tensorflow/issues/35887#issuecomment-679329549
www.youtube.com/watch?v=TfwjDgNoZYk
Hello Friends, Stay tuned and enjoy Machine Learning !!! Cheers !!! #freecourses #kaggle #machinelearning Connect with me, ☑️ YouTube : https://www.youtube.com/c/DataMagic2020 ☑️ Facebook : https://www.facebook.com/datamagic2020 ☑️ Instagram : http://instagram.com/datamagic2020 ☑️ Twitter : http://www.twitter.com/datamagic5 ☑️ Telegram: https://t.me/datamagic2020 For Business Inquiries : datamagic2020@gmail.com Best book for Machine Learning : https://amzn.to/3qCe0Rf 🎥 Playlists : ☑️Machine Learning Basics https://www.youtube.com/playlist?list... ☑️Feature Engineering/ Data Preprocessing https://www.youtube.com/playlist?list... ☑️OpenCV Tutorial [Computer Vision] https://www.youtube.com/playlist?list... ☑️Machine Learning Algorithms https://www.youtube.com/playlist?list... ☑️Machine Learning Environment Setup https://www.youtube.com/playlist?list... ☑️Machine Learning Model Deployment https://www.youtube.com/playlist?list... ☑️Machine Learning Projects https://www.youtube.com/playlist?list... ☑️Kaggle Tutorial https://www.youtube.com/playlist?list... ☑️Microsoft Lobe Tutorial https://www.youtube.com/playlist?list... Thank you for watching !! Please Like, Comment, Share and Subscribe!!! This episode can answer below queries:
youtube.com/playlist?list=PLBrGAFAIyf5rby7QylRc6JxU5lzQ9c4tN
OS 강의를 추천 드리고 싶어서 글 올립니다.
한국기술교육대학교의 김덕수 교수님 수업입니다.
한편 한편 분량도 적당하면서, 너무나도 쉽고 친절하게 설명을 해주셔서 이해 시켜주는 명강이라고 생각합니다.
저도 얼마 전에 완강했는데, 임베디드 주니어 개발자로서 배우는 것이 정말 많은 수업이었습니다. 수업 내용이 곧 실무랑 직결 되는 만큼 실용성도 높은 수업이라고 생각합니다!
프로세스 스케쥴링 알고리즘,
메모리 관점에서 지역성(locality)과 이를 이용한 성능 개선 방법,
멀티 프로세스 환경에서 뮤텍스 락이 왜 필요한지, <-> 데드락
Context switch를 피해야 하는 이유
Physical, Virtual 메모리 관리 방법 및 알고리즘
기타 등등..!
물론 필수 교양으로 모두들 잘 알고 계시는 내용 이겠지만, 한번 더 추천 드립니다!
[자기주도온라인평생학습] SQL 리스트 - 30개 교육과정(WITHMOOC) SQL for Data Science Capstone Projec (0) | 2020.03.19 |
---|
딥러닝 기본과 NLP를 익히는데 도움이 될 만한 최신 (2020년 2021년) 동영상 강좌 13종입니다.
하나 하나 직접 들어본 분의 추천이니 관심 있으신 분들은 보시면 좋을 듯 합니다.
1. Deep Learning: CS 182 Spring 2021
Includes a great introduction to deep learning starting with the machine learning basics moving into more core topics like optimization. (by Sergey Levine)
2. Deep Learning (with PyTorch)
This is one of the most recent deep learning courses focusing on hot topics like self-supervised learning, transformers, and energy based models. (by Alfredo Canziani)
3. Deep Learning Crash Course 2021
This course is focused on the popular free book available on the d2l.ai website. If you have been studying the book, this set of lectures will come in handy. (by Alex Smola)
4. Natural Language Processing
If you are not too familiar with natural language processing (NLP) concepts, this is a great place to start. It provides short and accessible summaries of some of the most important techniques used to solve NLP problems. (by Machine Learning University)
5. CMU Neural Nets for NLP 2021
This course covers topics related to how neural networks are used in natural language processing (NLP). (by Graham Neubig)
6. CS224N: Natural Language Processing with Deep Learning
This has been one of the most popular NLP courses for some time now. It focuses on the use of the latest deep learning techniques applied to NLP problems. (by Chris Manning)
7. fast.ai Code-First Intro to Natural Language Processing
The NLP courses above focus heavily on the theory. To get the practical side of NLP, this fast.ai course will be a great place to start. (by Rachel Thomas)
8. CMU Multilingual NLP 2020
Graham Neubig also provides another great course that focuses on multilingual NLP. Topics range from data annotation to code switching to low resource automatic speech recognition. (by Graham Neubig)
9. Deep Learning for Computer Vision 2020
This course focuses heavily on the latest techniques in deep learning for computer vision tasks. From attention mechanism to generative models. (by Justin Johnson)
10. Deep Reinforcement Learning: CS 285 Fall 2020
Focuses on the use of deep learning-based architectures for reinforcement learning problems. (by Sergey Levine)
11. Full Stack Deep Learning 2021
While most of the courses above focus heavily on theory, this course specifically focuses on the ecosystem of tools used to develop and deploy deep learning models. (by Josh Tobin, Pieter Abbeel, Sergey Karayev)
12. Practical Deep Learning for Coders
This is another course by fast.ai focusing on a coder-first approach to deep learning. (by Jeremy Howard)
13. Applied ML
This is an ongoing course teaching how to build a product grade product through ML techniques and tools. (by Made with ML)
https://www.facebook.com/groups/KaggleKoreaOpenGroup/permalink/863920357673398/
캐글 코리아 (Kaggle Korea) : 안녕하세요! 이번에 끝난 캐글 대회 RANZCR CLiP - Catheter and Line Position Chall
안녕하세요! 이번에 끝난 캐글 대회 RANZCR CLiP - Catheter and Line Position Challenge 에서 11위/1547명 으로 솔로 금메달을 따게 되어서, 간단하게 대회 리뷰해보고자 글을 올리게 되었습니다 :) 1. 대회
www.facebook.com
안녕하세요! 이번에 끝난 캐글 대회 RANZCR CLiP - Catheter and Line Position Challenge 에서 11위/1547명 으로 솔로 금메달을 따게 되어서, 간단하게 대회 리뷰해보고자 글을 올리게 되었습니다 :)
1. 대회 소개
chest x-ray 이미지를 인풋으로 받아, 환자에게 삽입된 카테터(튜브)의 종류 및 올바른 위치에 삽입됐는지의 여부를 분류하는 multi-label classification 문제입니다. classification 레이블 뿐 아니라, 일부 이미지에 대해서는 카테터의 위치 정보가 주어졌습니다. (첨부한 두 번째 그림 참고 - 같은 색의 점들을 이으면 카테터의 위치가 됩니다.)
2. 솔루션 https://www.kaggle.com/c/ranzcr-clip-catheter-line-classification/discussion/226557
1) 고해상도를 어떻게 활용할 것인가? - Downconv
2048x2048 이상의 높은 해상도의 이미지가 주어졌습니다. 그러나 이 이미지를 바로 인풋으로 넣으면 GPU 메모리가 감당하지 못하고, 그렇다고 해서 작게 resizing해서 넣으면 정보를 잃어버립니다.
Conv2d 레이어 하나를 이용해 2048->1024로 이미지를 변환하는 downconv를 도입해 이를 해결했습니다. avg pooling한 이미지를 이 downconv의 아웃풋과 concat해서 CNN의 인풋으로 넣었습니다.
(첨부한 세 번째 그림 참고)
2) 카테터 위치 정보를 어떻게 활용할 것인가? - Pre-training
카테터 위치 정보는 단순 분류 레이블보다 공간적인 측면에서 훨씬 더 많은 정보를 가지고 있기 때문에 모델이 이를 활용할 수 있도록 하는 것이 중요했습니다. 따라서 저는 encoder에 추가적으로 classification head를 부착한 UNet 구조를 사용하였습니다.
카테터 위치 정보를 segmentation mask 형태로 전처리해, 이를 타겟으로 UNet을 학습시켰고, pre-trained UNet encoder를 가지고 classification 학습을 시켰습니다.
(첨부한 첫 번째 그림 참고)
3) unlabeled data를 어떻게 활용할 것인가? - Pseudo-training
주어진 labaled 데이터셋 말고, 외부 x-ray 데이터셋들이 많이 존재합니다. 이런 데이터셋들은 카테터 레이블링이 되어있지 않습니다. 최근 unlabaled 데이터셋을 이용한 semi-supervised 및 self-supervised learning들이 제안되고 있습니다.
제 경우 pseudo-labeling 후 재학습시키는 방법(pseudo-training이라고 우선은 명명하겠습니다)이 효과를 발휘했습니다.
(첨부한 네 번째 그림 참고)
3. 1위 솔루션 https://www.kaggle.com/c/ranzcr-clip-catheter-line-classification/discussion/226633
* 카테터 관련 마스크 3개 생성, 이를 예측하는 UNet 모델 여러개 학습 후 pseudo-training
* 원래 이미지에 예측된 마스크 3개를 concat한 것을 인풋으로 사용하여 분류 모델 여러개 학습 후 pseudo-training
* multi-label 중 적절한 경우 multi-class loss 적용
* segmentation 이미지 사이즈 > 1024, classification 이미지 사이즈: 384~512 (segmentation에서 훨씬 높은 이미지 해상도를 필요로 함을 알 수 있습니다.)
4. 느낀점
* 아이디어를 실험해볼 때, 세부 구현 방법에 따라 그 결과가 달라지는 경우가 있는 것 같습니다.
* 고해상도, 큰 모델일수록 성능이 올라가는 이미지 대회는 특히 GPU/TPU장비가 중요한 것 같습니다. 4 x RTX3090 & 쓰레드리퍼3990x 서버를 제공해주시고, 대회 막판에 추가 서버들도 쓸 수 있게 해주신 Upstage 에 감사의 말씀 드립니다 :)
* 여담으로, 이번 대회에서 실험한 로그들을 살펴보니, 280개의 실험을 했고, 60여개의 학습 옵션을 만들었고, 여러 실험들을 하는 데 사용된 학습시간은 총 1051시간이네요. 제가 아직 실력이 부족해서인지, 시행착오가 많았던 것 같습니다..ㅎㅎ
많이 부족한 글 읽어주셔서 감사합니다!
[ TF Everywhere 행사 영상 및 메이킹 영상 공유]
안녕하세요! 어제 날짜로 TF Everywhere 텐플마을에 오신것을 환영합니다 행사가 성공적으로 마무리 되었습니다. 와-아! 게더타운부터 유튜브 스트리밍까지 700🔥이 넘는 뷰를 달성하며 많은 분들께서 관심을 가져주셨는데요. 👾게더 타운에서 사과찾기, 9와 3/4 공간 찾기👾등 팝업이벤트도 너무 빠르게 성공 해주셔서 역시 개발자 컨퍼런스 답다~ 싶게 열정이 뿜뿜!! 저도 많이 배워간 행사였습니다.
(본론) 유튜브 스트리밍으로 각 세션을 참관하셨지만 안타깝게 게더 타운 티켓을 겟하지 못하신 분들을 위해! 이 행사에 대해 짤막하게 메이킹 영상을 만들어보았습니다! 더불어 병렬적으로 진행된 네가지 세션에 대한 업로드 영상도 함께 공유하니 현장에서 멀티 세션을 모두 보지 못해 아쉬웠던 분들 역시 보시면 좋을 것 같네요 :)
모든 스피커분들 감사드리고, 행사에 큰 도움 주신 권순선, 김나연 님 너무 감사드립니다! 좋은 주말 되세요!
- Beginner Session: https://youtu.be/2QxfO_md9N4
- Developer Session: https://youtu.be/Xe_gFoVTwcQ
- Competition Session: https://youtu.be/xgt7FKfpF2Q
- Tutorial&Guide Session: https://youtu.be/9Y5TunPY-xg